TRANSFAC 2.0
Discover
TF binding sites in promoters
and enhancers of your genes
Reconstruct
signal transduction network
controlling your genes
signal transduction network
controlling your genes
Identify
drug targets
and disease biomarkers
drug targets
and disease biomarkers
Discover
TF binding sites in promoters
and enhancers of your genes
Reconstruct
signal transduction network
controlling your genes
Identify
drug targets
and disease biomarkers
drug targets
and disease biomarkers
Discover
TF binding sites in promoters
and enhancers of your genes
Reconstruct
signal transduction network
controlling your genes
Identify
drug targets
and disease biomarkers
TRANSFAC PATHWAYS
Reconstruct signal transduction network controlling your genes
Introduction
TRANSFAC PATHWAYS package comprises everything of TRANSFAC BASIC package plus TRANSPATH® database which is the comprehensive signal transduction database of mammalian signal transduction and metabolic pathways.
As one of the earliest pathway databases ever created, it has grown since to the remarkable volume of more than 1,200,000 manually curated reactions. One of the largest pathway databases available, optimally suited for geneXplain’s proprietary Upstream Analysis.
Database content
TRANSPATH organizes the information about genes/molecules and reactions according to multiple hierarchies. Its sophisticated structure makes it one of the scientifically best conceptualized pathway resources, suitable for multi-purpose uses. It is complemented by one of the richest corpora of pathway data available among all public domain and commercial sources, all manually curated by experts.
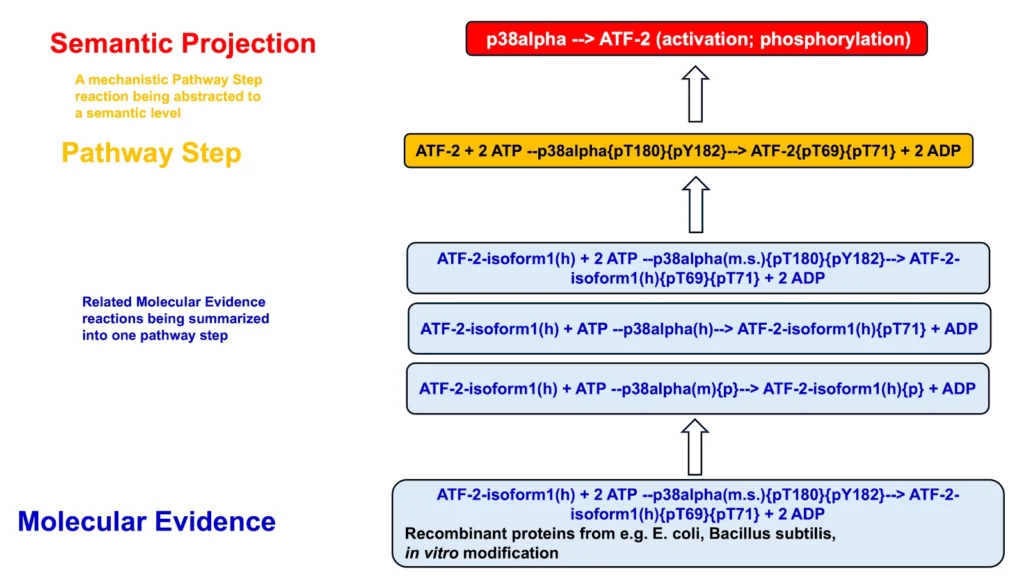
Reaction hierarchy in the TRANSPATH® database on molecular pathways.
Individual reactions are documented with all experimental details, in a strictly mechanistic way that includes all reaction partners and the taxonomic origin of each molecule as reported in the published experiment (“molecular evidence level”). All evidence for a certain pathway step is accumulated to provide a more comprehensive and complete picture (“pathway step level”). On top, a semantic view is provided, which focuses on the key components only and omits mechanistic details as well as small abundant molecules (“semantic projection”). Complete networks and pathways are built from molecules and their reactions.
To consider the heterogeneity of information given in the original publications, TRANSPATH transparently but precisely differentiates protein molecules according to:
their relatedness within one genome
Information can be specifically retrieved regarding:
(a) specific individual proteins,
(b) all products of a certain gene (isoforms),
(c) different family relation levels (e.g., paralogs);
their relatedness between different genomes (orthology)
their association and modification status
(a) protein complexes are specified with their exact composition;
(b) post-translational modifications are given with their exact positions in the protein.
Pathways:
TRANSPATH® database collects and systematize canonical signal transduction and metabolic pathways. Currently it collects over 1500 various pathways. An example of one of such pathway “TGFbeta network” is shown below. We record a precise information about molecular details of the pathway reactions including posttranslational modifications of the molecules involved, their complexes and isoforms.
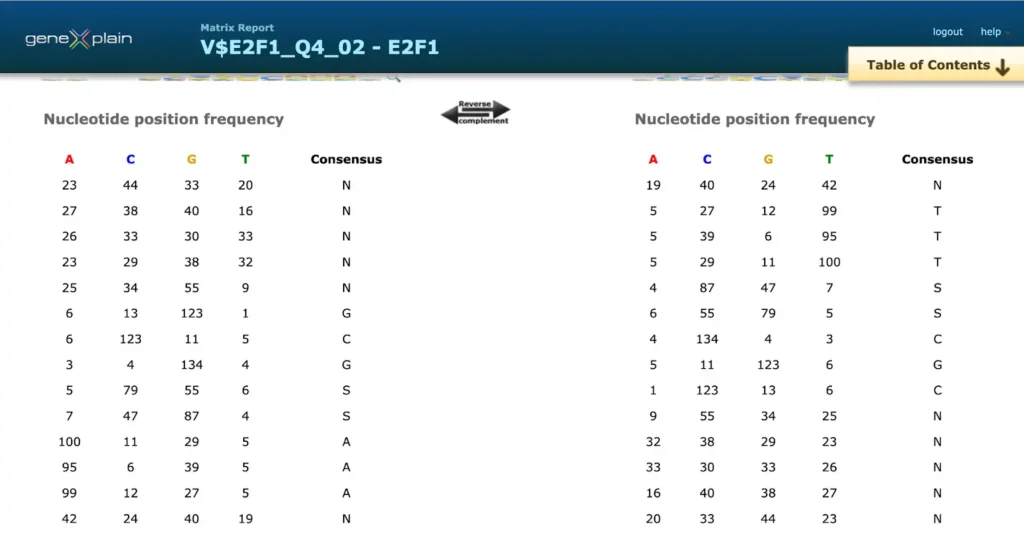
Visualization of the TGFbeta network with the geneXplain platform; data were retrieved from the TRANSPATH® database.
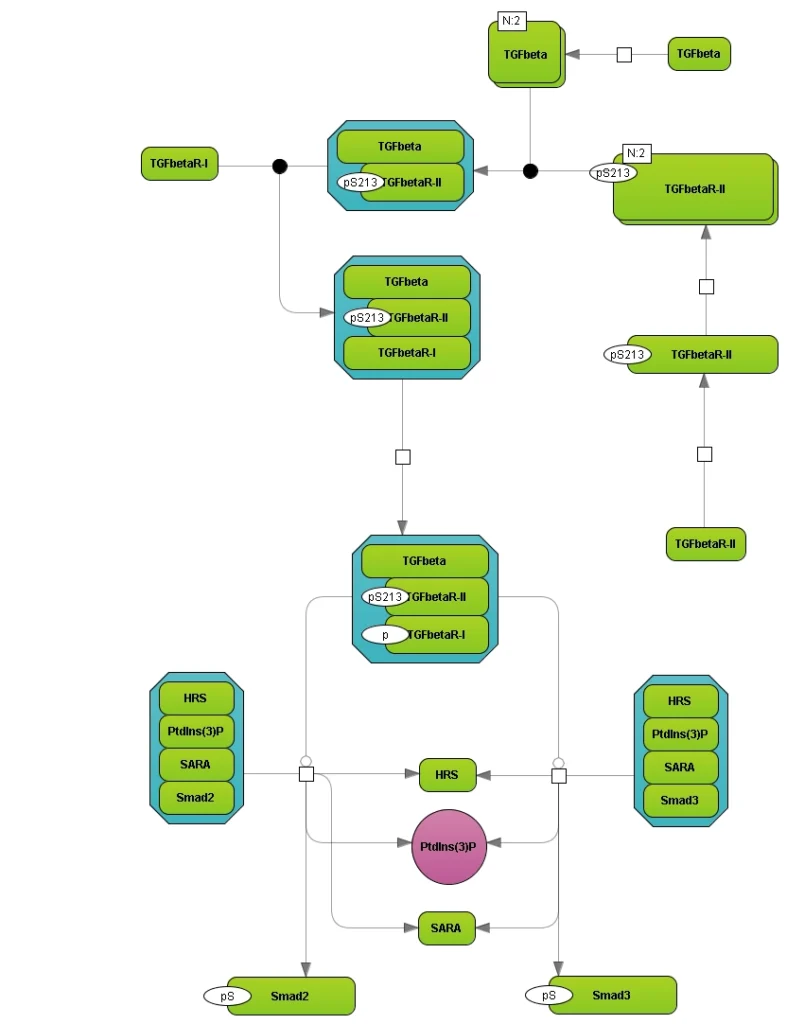
For each of the pathways contained in TRANSPATH database, users find explicit information on the pathway subcomponents and individual reactions, from which the pathway subcomponents are assembled. Below is a fragment of the detailed description of the IFNalpha/beta pathway.
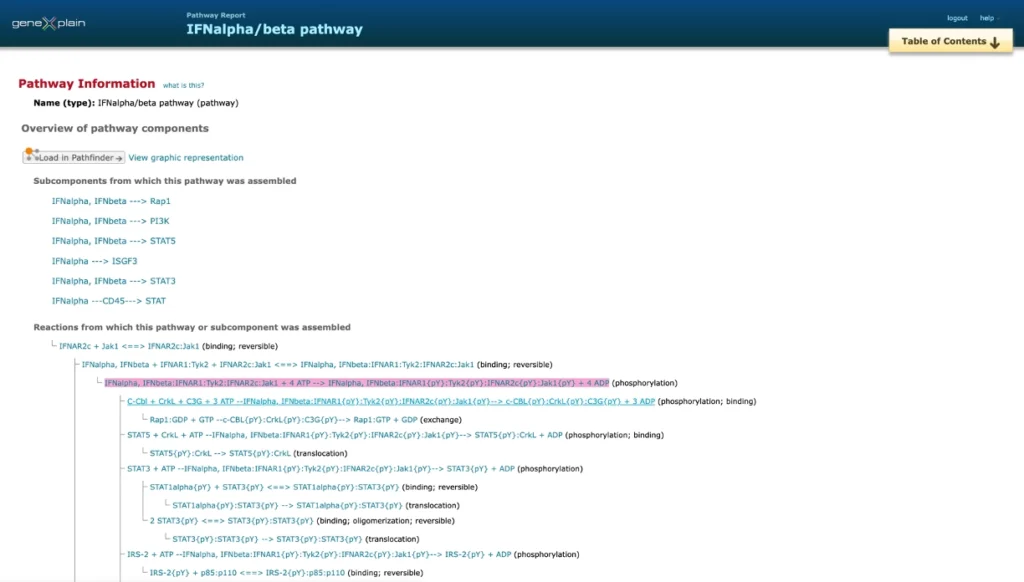
Reaction network:
TRANSPATH® database is one of the most comprehensive repositories of signal transduction reactions in mammalian cells. It collects over 1,200,000 experimentally proven reactions of phosphorylation, acetylation, ubiquitination, translocation and other types of reactions involved in signal transduction. These reactions build a highly connected regulatory reference network which is used for analysis and reconstruction of molecular mechanisms of diseases, identification of master-regulators and drug targets.
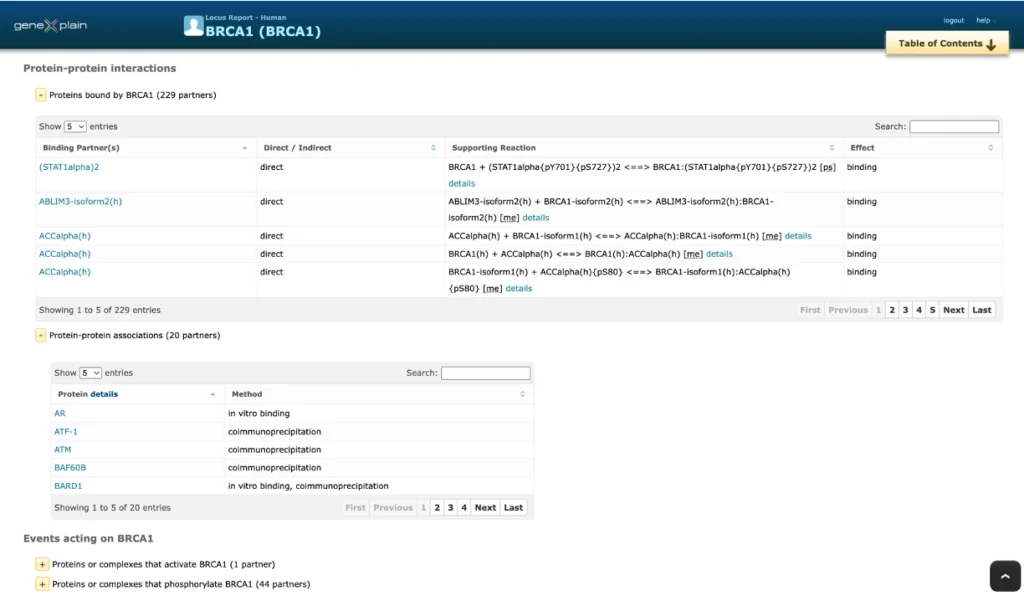
The database also contains the protein-protein interactions (PPIs) information, as well as information of post-translational modifications (PTMs):
Get free Match demonstration videos .
Get current statistics of TRANSPATH database
Tools
Pathways analysis
What is Pathway Analysis?
This may have different flavors:
Therefore, the geneXplain platform provides an empirical way to identify the specific combination of sites that characterizes a given set of co-regulating promoters.
Most commonly, it is of interest to know which signaling or metabolic pathways are activated under certain experimental conditions.
A slightly different question may be to find out which pathways were used to express a certain observed phenotype.
Both types of problems can be conveniently addressed with the geneXplain platform.
Approaches to pathway analysis
To find out whether among all genes induced in an experiment those are overrepresented that encode components of a certain pathway, conventional gene set enrichment analysis (GSEA) and related methods can be applied. In such an approach, however, topological information about the pathway is lost.
More sophisticated is to search for those networks, pathways or paths where many linked components have been induced. This is provided by the platform option “Cluster by shortest path”. A visualization of differential expression onto a known pathway is shown in the figure below. These known pathways may be documented in the databases TRANSPATH® (manually curated information; example shown) or GeneWays (compiled by text mining).
Learn more about the geneXplain platform.
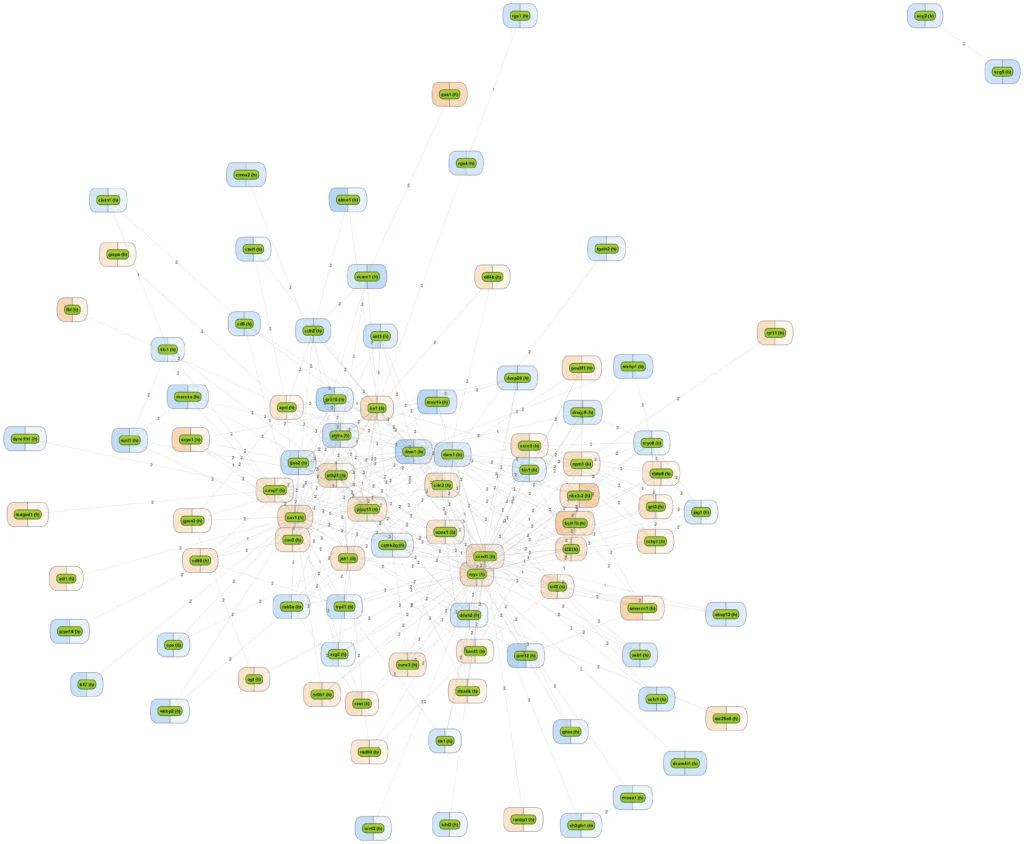
When starting from a set of differentially expressed genes or their products, resp., it is frequently of interest to see what is their common activator. Such convergence points of upstream pathways are potential master regulators, or key nodes.
The next figure shows how the upstream paths of a set of proteins (blue) converge in one master regulator (here: AKT1, red). The database behind this analysis is TRANSPATH®. It can be seen how a section of the whole pathway (overview in the lower right window) is amenable to editing in the main work area. Detailed information about a selected component, like the mTor complex in this example, are displayed in the Info Box at the lower left corner.
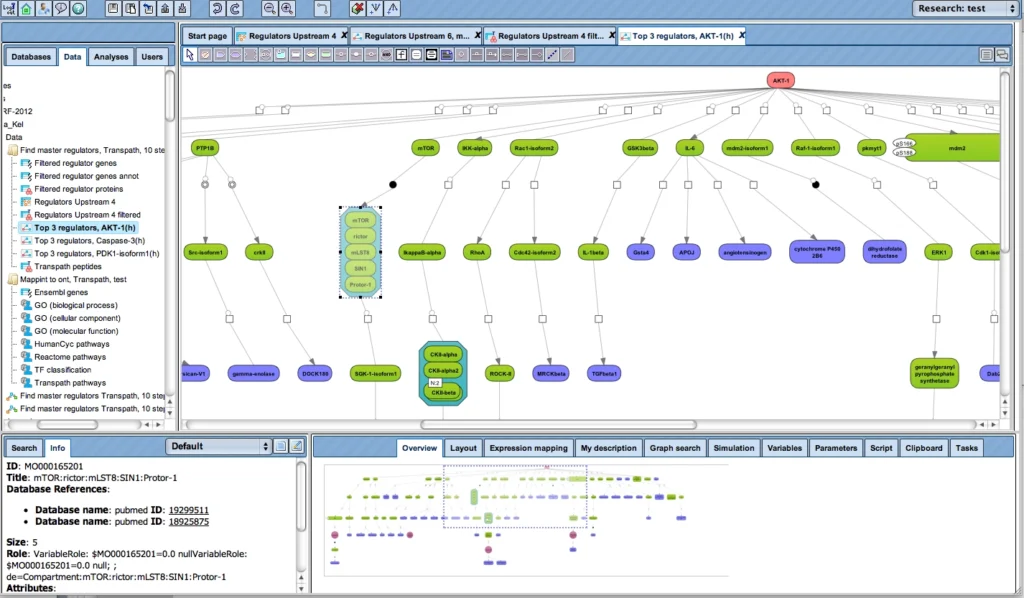
This type of analysis can be combined with the visualization of differentially expressed genes and their expression behavior, in the same way as shown above.
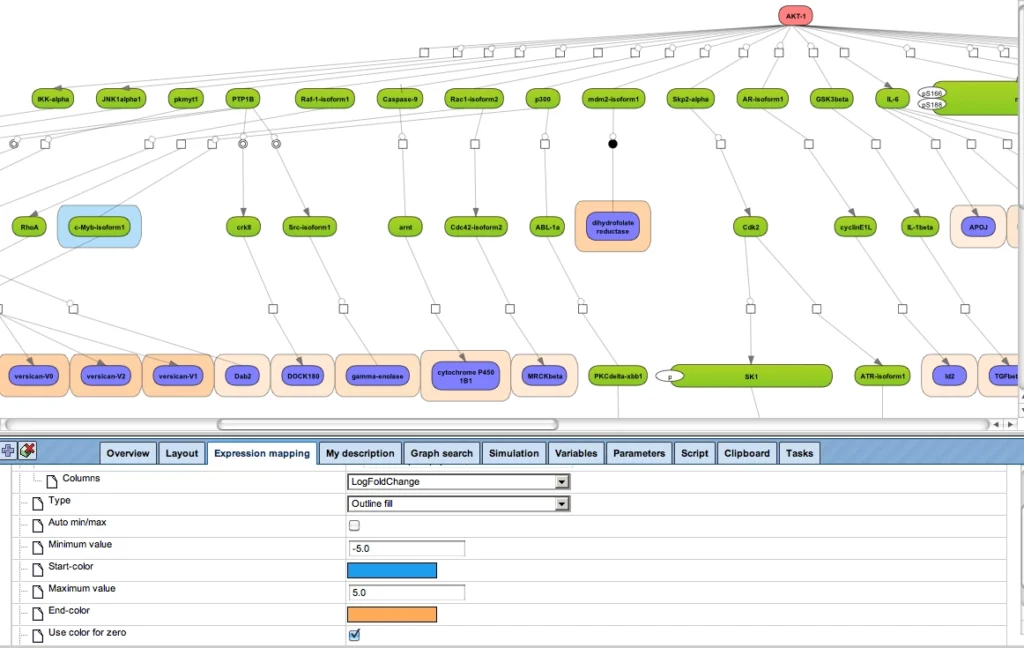
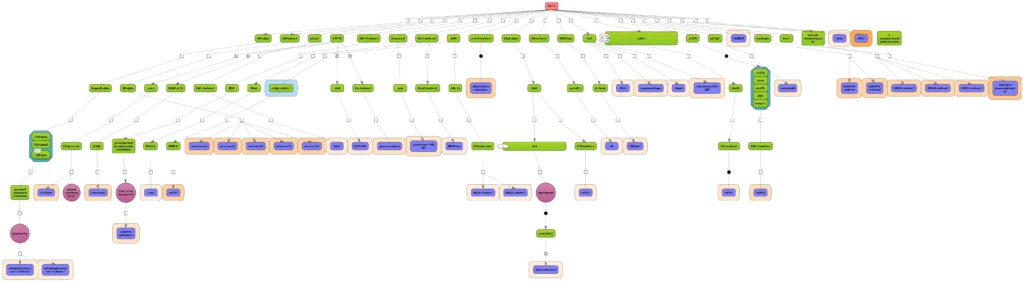
Graph layout
Proper handling of the layout is a particular challenge when displaying networks. The implementation in the geneXplain platform ensures an easy and fast reorganization of the layout between a hierarchical, force-directed and orthogonal layout scheme.
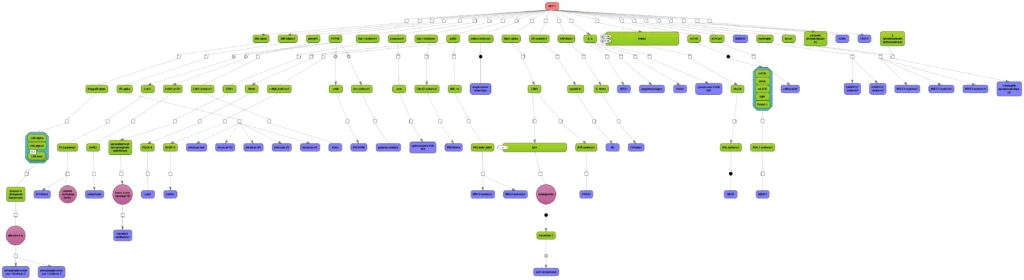
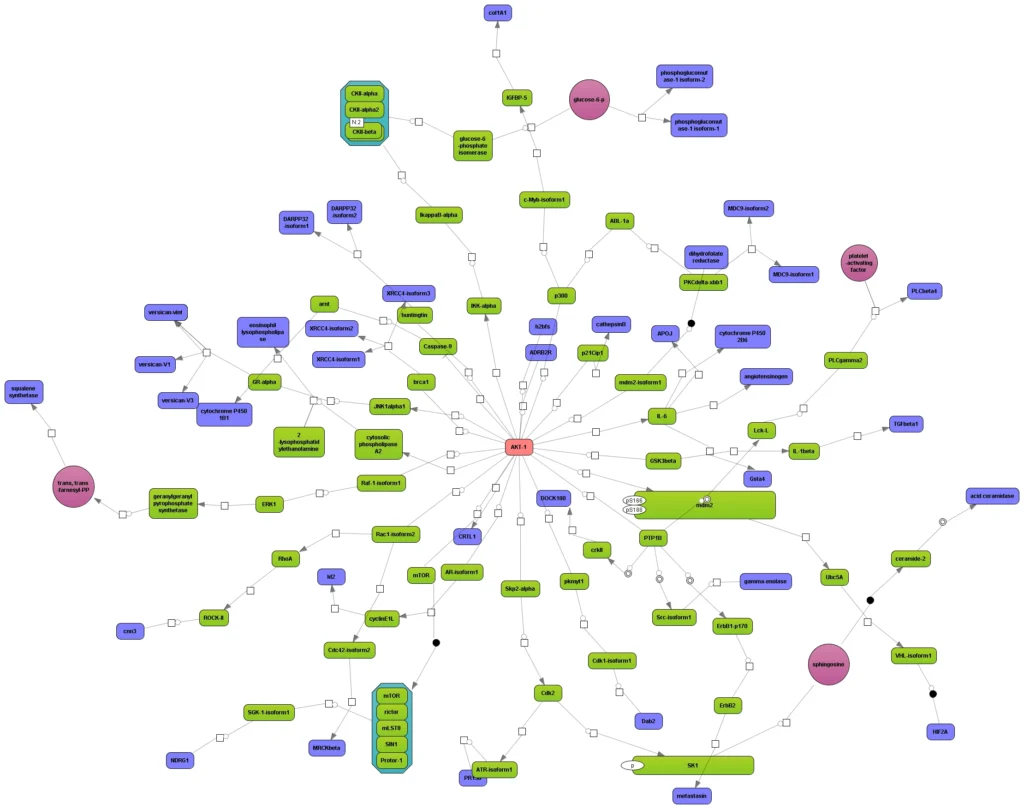
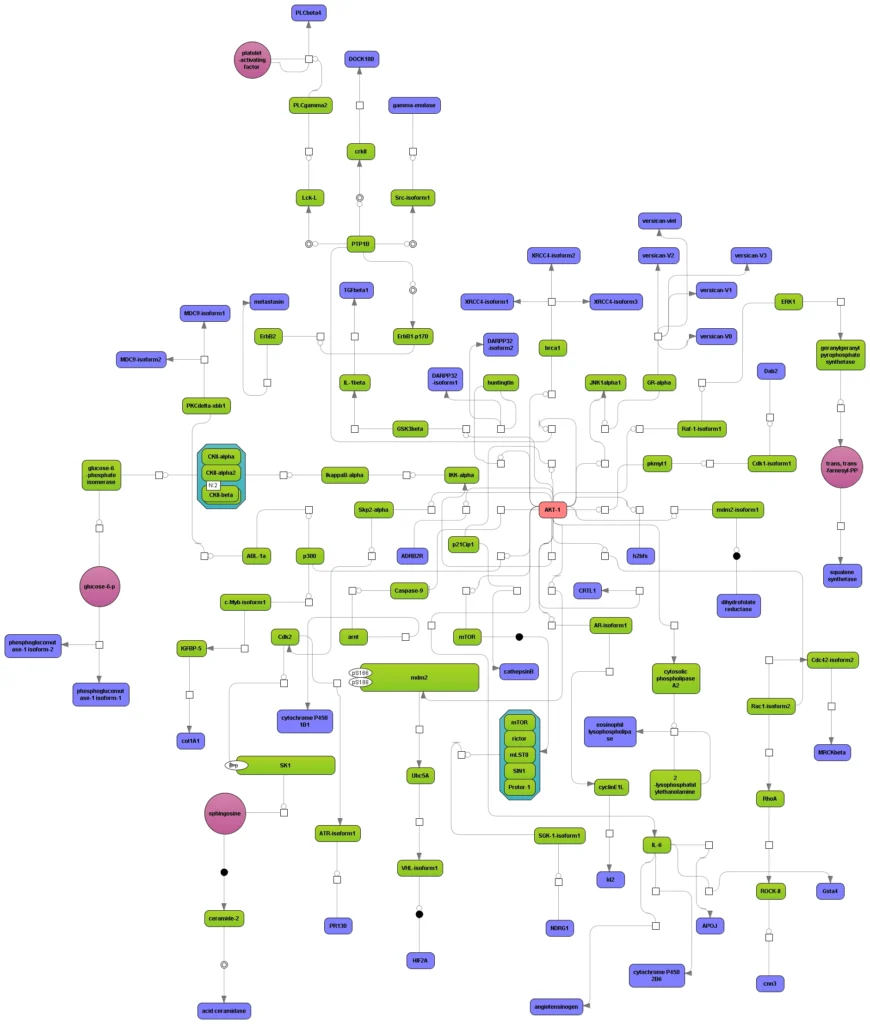
Graph search
Any diagram constructed from TRANSPATH or GeneWays contents can be manually expanded to molecules that are connected to a selected node (see figure below). Subsequent automatic redesign will refine the appearance of the graph according to the chosen layout style.
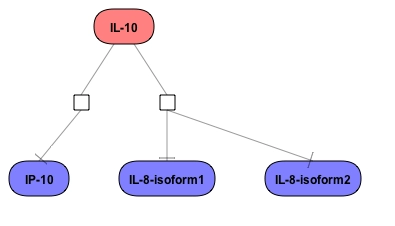
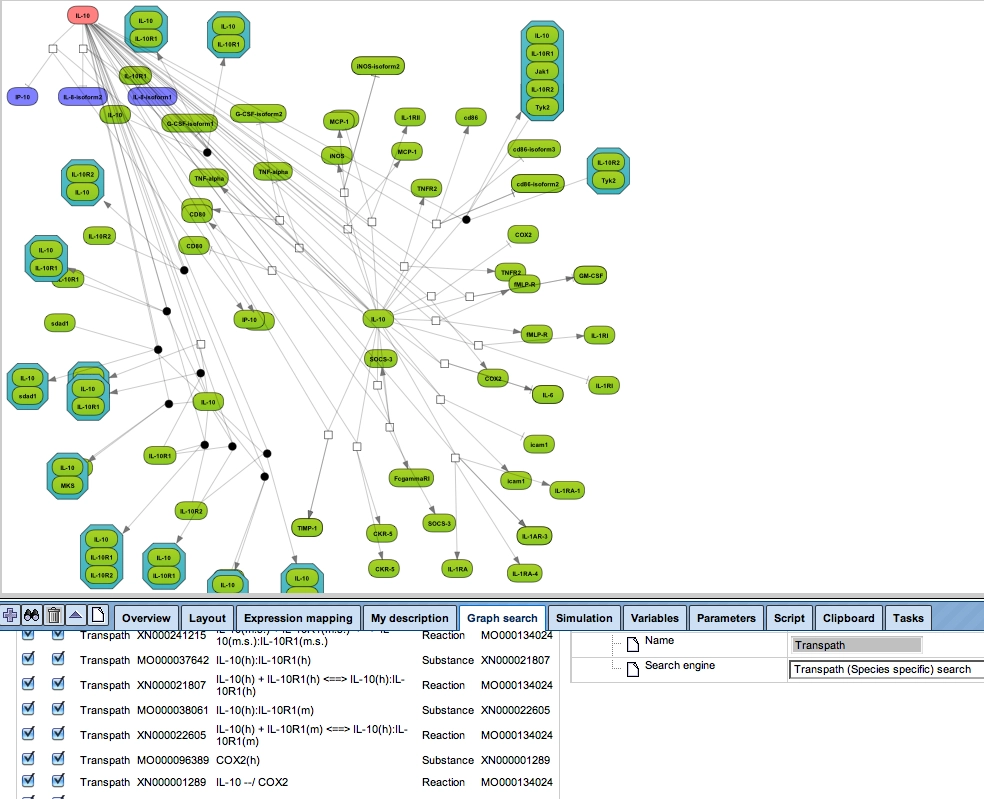
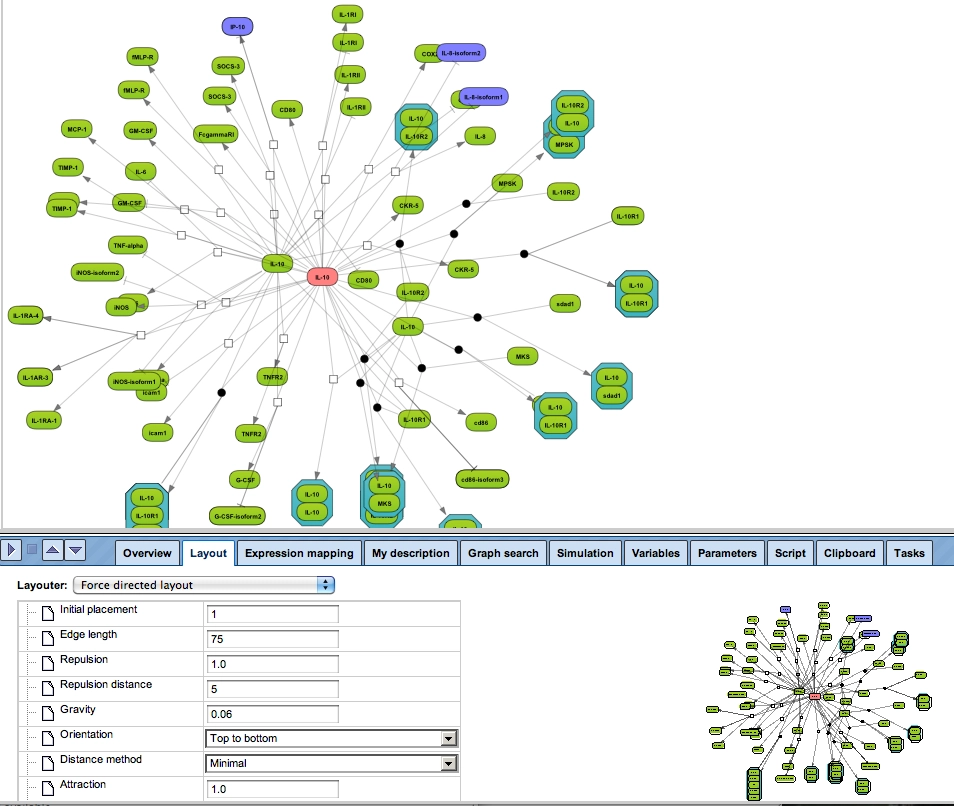
Joining graphs
Several diagrams, e.g. pointing at different master regulators (figure below, red nodes), can be easily joined.
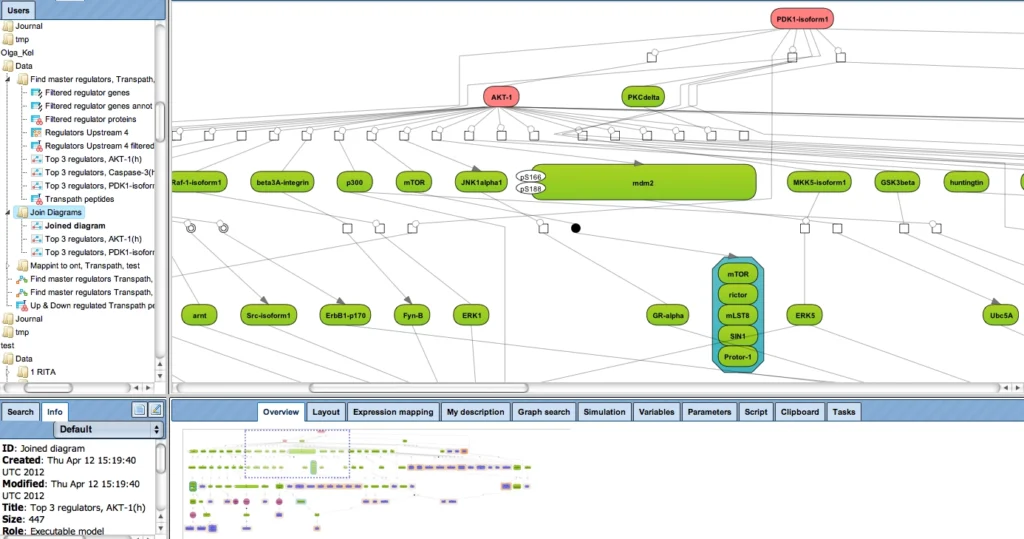
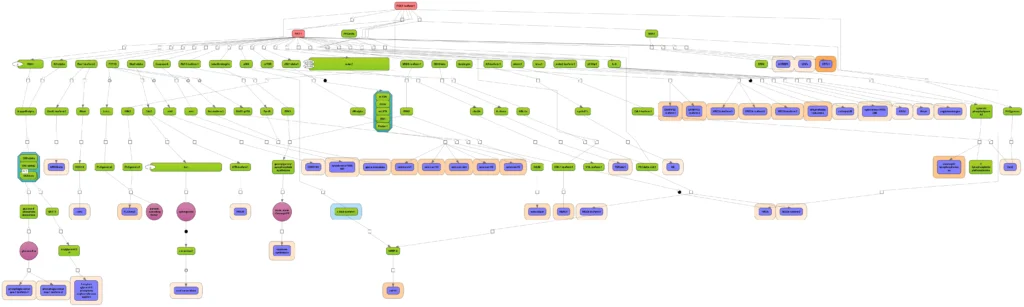
SBGN viewer:
- PathFinder is the web browser tool for visualization of signaling and metabolic pathways using (System Biology Graphic Notation) SBGN standard.
Upstream analysis:
The TRANSFAC PATHWAYS package uniquely combines promoter analysis with pathway analysis, enabling the identification of master regulators in gene regulatory networks. No other tool on the market provides such an integrated capability.
ODE modeling:
- The TRANSFAC PATHWAYS package includes geneXplain platform modeling tools on the basis of BioUML simulation environment, which is according to the independent study (Maggioli F., Mancini T., Tronci E. SBML2Modelica: Integrating biochemical models within open-standard simulation ecosystems. Bioinformatics, 2019, doi: 10.1093/bioinformatics/btz860) shown as “the best and fastest SBML simulation engine”. In direct comparison to other simulation engines, such as CAPASI, SystemModeler, SMBL2Modelica and others, BioUML shows the fastest simulation time among 613 curated models of BioModels database. Also it was shown that only BIoUML with its powerful simulation engine that supports ODE, DAE, hybrid,1D PDE passes 100% of simulation tests out of SBML Test Suite Core v3.3.0.
The BioUML simulation environment also provides a wide range of instruments for visual modeling including network modeling, composite modeling; and agent-based modeling.
BioUML web GUI for visual modeling
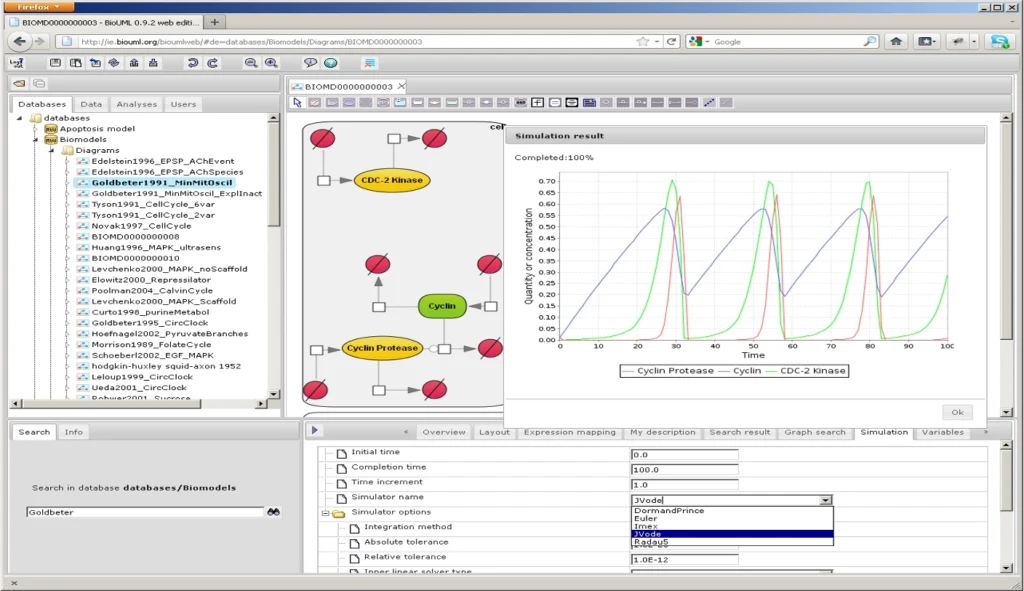
BioUML parameter fitting engine supports: fitting to time courses or steady states; multi-experiment-fitting; constraint optimization; local/global parameters; and parameters optimization using java script. The following optimization methods are implemented in BioUML: Adaptive simulated annealing, Evolutionary programming, Particle swarm, Stochastic ranking evolution strategy, and cellular genetic algorithms.
An integrated apoptosis model build using TRANSFAC PATHWAYS:
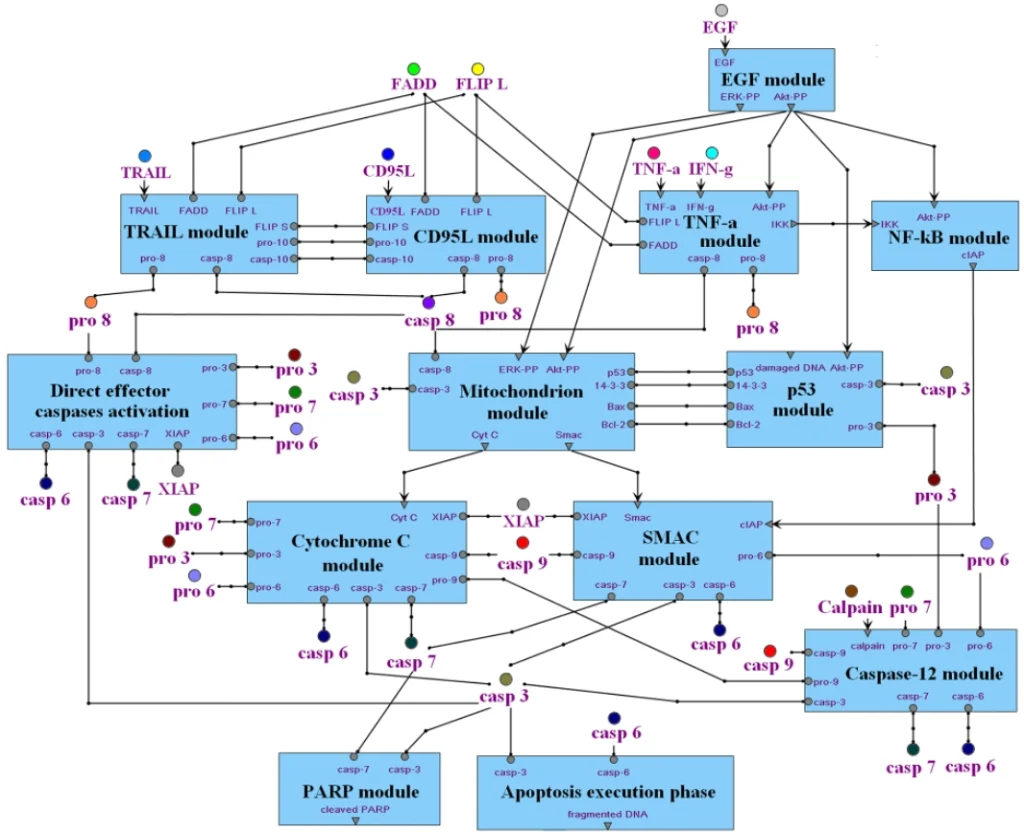
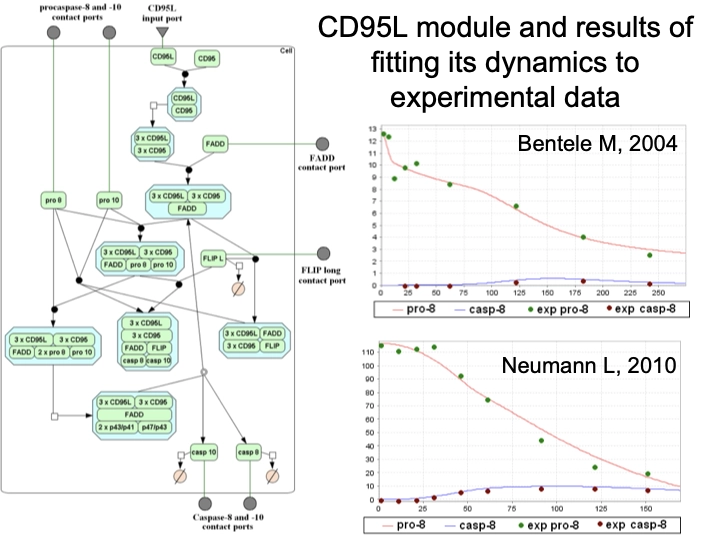
TRANSFAC DISEASES
Identify drug targets and disease biomarkers
Introduction
TRANSFAC DISEASES package comprises everything of TRANSFAC BASIC and TRANSFAC PATHWAYS packages plus The Human Proteome Survey Database (HumanPSD) which is a catalog of proteins and their complexes from human cells, plus their orthologs from mouse and rat sources.
Its main focus is on the association of human proteins with diseases as well as on their potential use as biomarkers.
TRANSFAC DISEASES package also includes Genome Enhancer – a fully automated pipeline for patient omics data analysis, which identifies prospective drug targets and corresponding treatments by reconstructing the molecular mechanism of the studied pathology.
Database content
HumanPSD reports detailed information about the role of human proteins in diseases. Information can be retrieved on the molecular functions, biological roles, localization, and modifications of proteins, expression patterns across cells, tissues, organs, and tumors, consequences of gene mutations in mice, and the physical and regulatory interactions between proteins and genes.
Biomarkers:
- HumanPSD reports over 140,000 gene-to-disease biomarker associations (causal, correlative, preventive, negative, prognostic). Over 2,000,000 annotation lines with detailed description of the biomarkers manually curated from a wide spectrum of scientific literature and patents.
Below is the table of disease biomarker associations for the human gene TP53.
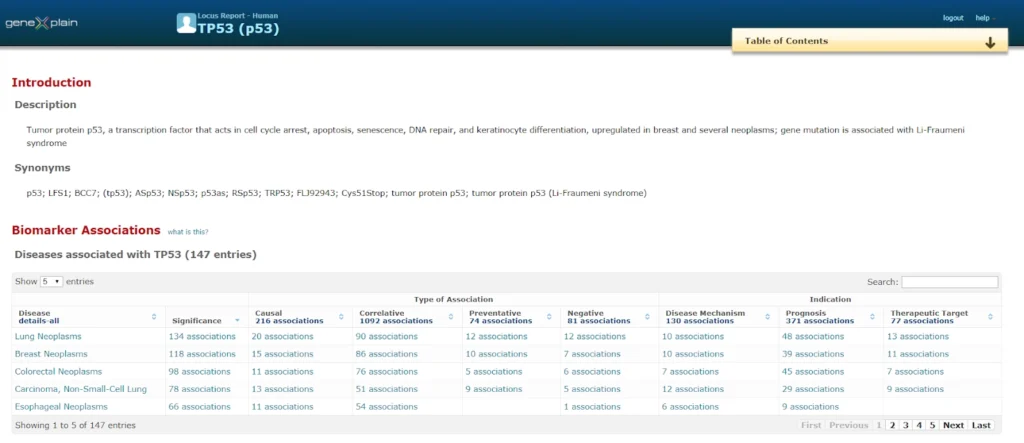
Example of full locus report on human TP53 gene
Disease mechanism:
- HumanPSD reports description of disease molecular mechanisms for over 3,900 human diseases.
Example of the full Disease report on Lung Neoplasms
HumanPSD also reports inferred disease-disease relationships on the basis of shared Causal and Preventative biomarker genes. Disease networks are derived from these relationships and organized into clusters with apparent biomedical relevance. The inferred disease-disease associations coincided with known clinical correlations such as comorbidities or known disease etiologies.
Adenocarcinoma
Disease similarity map:
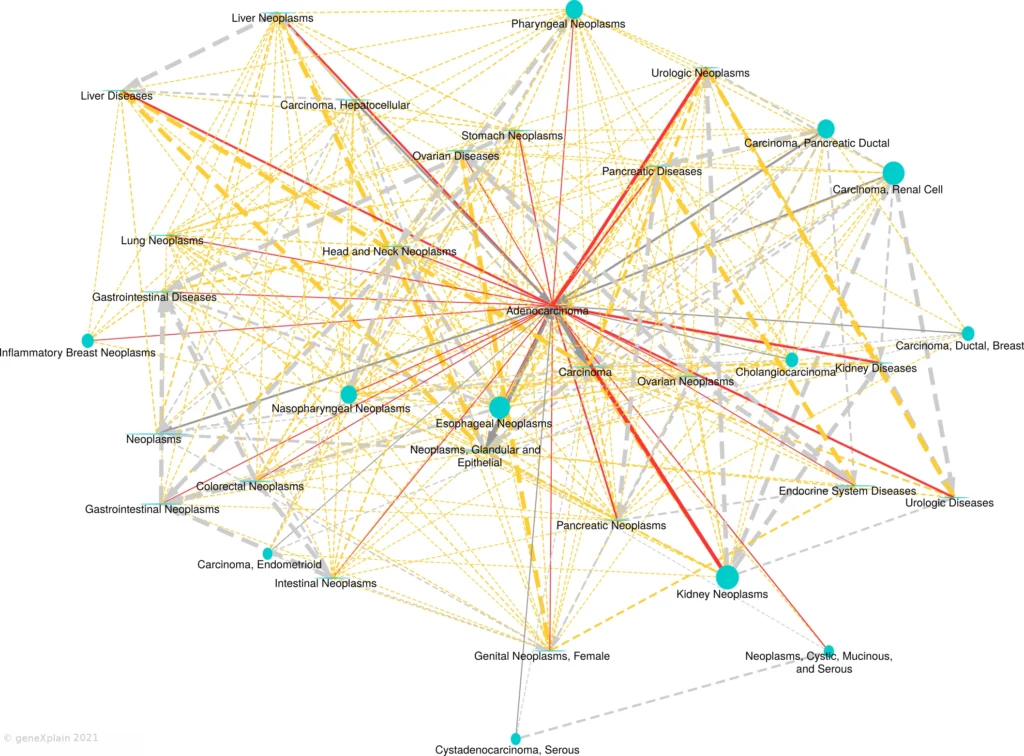
Legend:
This Disease Similarity Map connects diseases (nodes) with edges on the basis of common causal biomarker genes (edges are shown for FDR < 0.05 and overlap size >= 2). The primary disease is represented by a red diamond shape, neighboring diseases by blue circles. Solid edges connect the primary disease to neighboring diseases. Dashed edges connect neighboring diseases. Undirected solid red edges connect the primary disease in the center with similar diseases (neighbors), undirected dashed orange edges connect similar neighbors. Gray arrows point from a child disease to the parent disease according to the MeSH hierarchy. Edge widths are proportional to the statistical significance of association and node sizes are proportional to the number of causal biomarker genes of the disease.
The full list of disease networks in HumanPSD database
Drugs and targets
HumanPSD reports over 55,000 drug targets and associated with them over 10,000 drugs.
An example of the full Drug report on Methotrexate
Clinical trials:
- HumanPSD reports over 1,100,00 clinical trial – disease connections extracted from ClinicalTrials.gov and AACT databases, and also from the registries and data partners contributions to the OpenTrials project.
Here is a screenshot of the information about clinical trials for Lung Neoplasms:
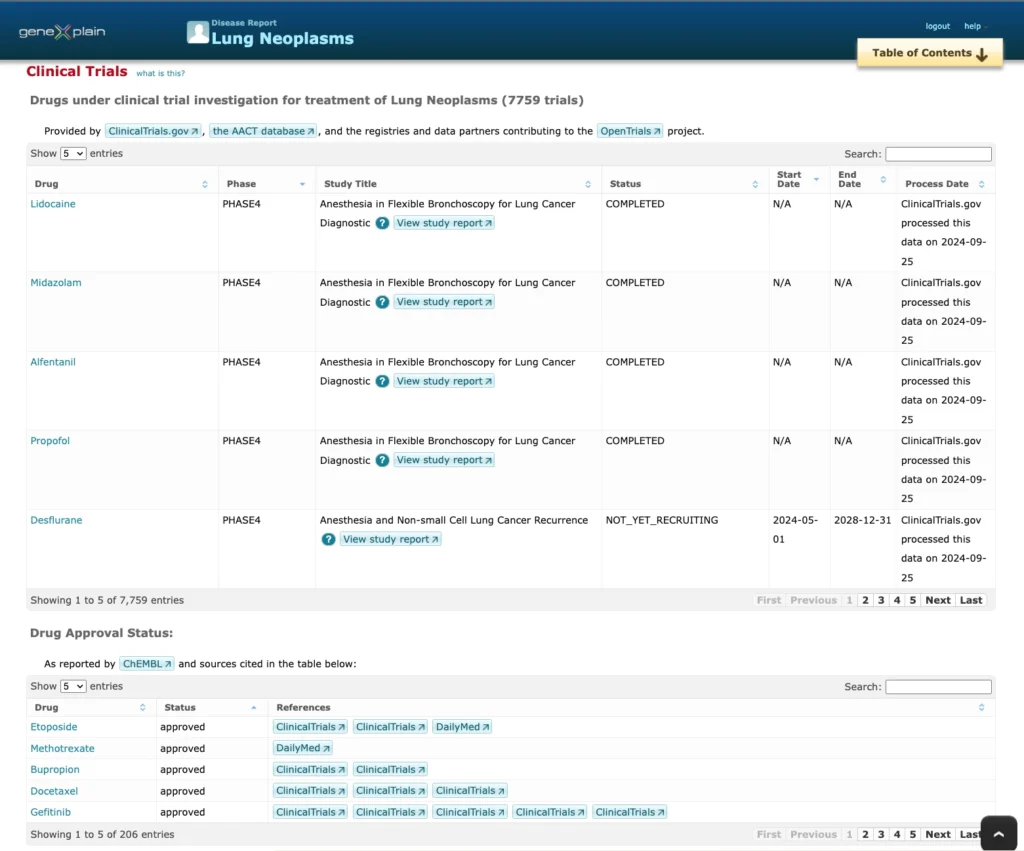
The full statistics of the HumanPSD and TRANSPATH databases
Tools
Genome Enhancer
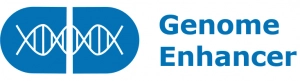
Welcome to the new era of Precision Medicine!
TRANSFAC DISEASES package includes an AI driven tool Genome Enhancer – a fully automated pipeline for patient omics data analysis, which identifies prospective drug targets and corresponding treatments by reconstructing the molecular mechanism of the studied pathology. Proven applications of Genome Enhancer include cancer, neurodegenerative diseases, infectious diseases, diabetes and metabolic diseases, hypertension.
Example Genome Enancer reports of data analysis and drug target prediction for: cancer, neurodegenerative diseases, infectious diseases, diabetes and metabolic diseases, hypertension.
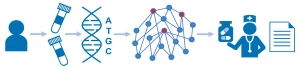
Genome Enhancer provides a powerful synergism between the automatic pipeline for multi-omics data processing and the comprehensive bioinformatics toolbox of the geneXplain® platform integrated with TRANSFAC, TRANSPATH, and HumanPSD databases.
Genome Enhancer offers:
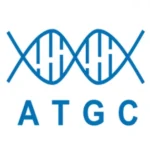
Multi-omics analysis
Use genomics, transcriptomics, metabolomics, proteomics, and epigenomics data in one analysis run and receive an integrated report
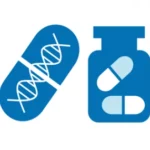
Personalized medicine
Running the analysis on omics data of a certain patient, you will identify personalized prospective drug targets and corresponding treatments
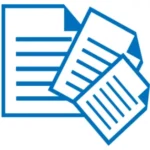
Scientific base
Integration of promoter and enhancer analysis with pathway reconstruction gives unrivaled disease molecular mechanism modeling accuracy
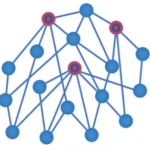
Drug target identification
Genome Enhancer reconstructs a complex network of signal transduction pathways that are activated in the pathology and identifies their key regulators
Genome Enhancer Algorithm
Genome Enhancer uses Upstream Analysis, an integrated promoter and pathway analysis, to identify potential drug targets of the studied pathology.
In the first step of this analysis the transcription factors that regulate differentially expressed or mutated genes are identified with the use of the TRANSFAC® database of transcription factors binding sites.
The second step searches for common master-regulators of the identified transcription factors by building a personalized signal transduction network of the studied pathology using the TRANSPATH® database of mammalian signal transduction and metabolic pathways. The identified master regulators are prospective drug target candidates. They are used for further selection of chemical compounds that can bring therapeutic benefit for the studied clinical case. In this step the HumanPSD™ database is employed to identify drugs that have been tested in clinical trials. The cheminformatic tool PASS predicts small molecules that can affect the identified targets.
Finally, Genome Enhancer generates a comprehensive analysis report about the personalized drug targets identified for a certain patient, or a group of patients, and the drugs that may be effective in this case. You can view a number of Genome Enhancer demo reports at the corresponding section of this page.
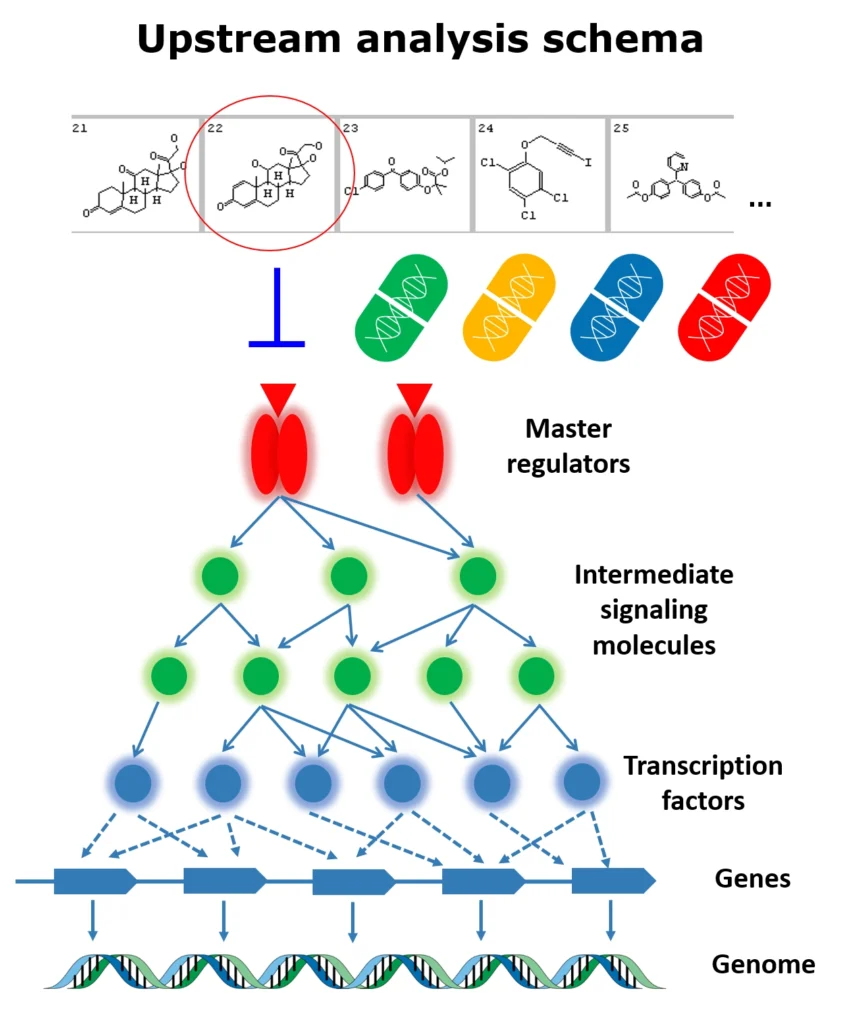
Check out the Genome Enhancer video channel run by the CSO of geneXplain GmbH Dr. Alexander Kel. See how various omics data can be analyzed in Genome Enhancer or even send your own data to Dr. Kel and he will show its analysis in one of the next videos!
Key features of the TRANSFAC DISEASES package tool – Genome Enhancer:
Disease mechanism:
Genome Enhancer applies AI algorithms such as Genetic Algorithm and complex graph analysis algorithms to discover disease molecular mechanisms and to identify potential drug targets. The analysis can be done in the context of over 3,900 different human diseases, including complex diseases such as cancer, cardio-vascular, auto-immune, neurodegenerative diseases as well as multiple genetic and rare diseases.
Reconstructed disease molecular mechanism of Glioblastoma tumors by comparing long-survival versus short-survival patient transcriptomics (RNA-seq data)
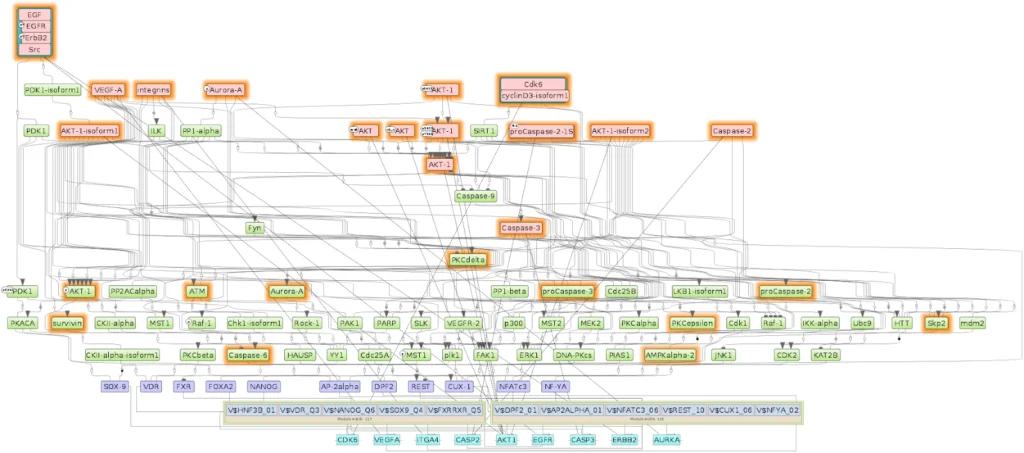
Multi-omics integration:
Genome Enhancer provides flexible integration of all five “-omics” data types: Transcriptomics, Genomics, Epigenomics, Proteomics, Metabolomics. Any combinations as well as individual omics data can be combined in one analysis run. The omics integration is done following the principles of organisation of molecular-biological and biochemical systems in eukaryotic cells. The Upstream Analysis integrates promoter and pathway analysis, to identify potential drug targets of the studied pathology. Transcriptomics data help to find differentially expressed genes (DEGs); Metabolomics help to reveal which of the DEGs are most critical for metabolome changes in the studied pathology; Epigenomic data help to identify most regulatory active genomic regions for searching for TFBS enrichment; Genomic data help to reveal TF binding sites affected by regulatory mutations; Proteomics data help to strengthen the master regulator search.
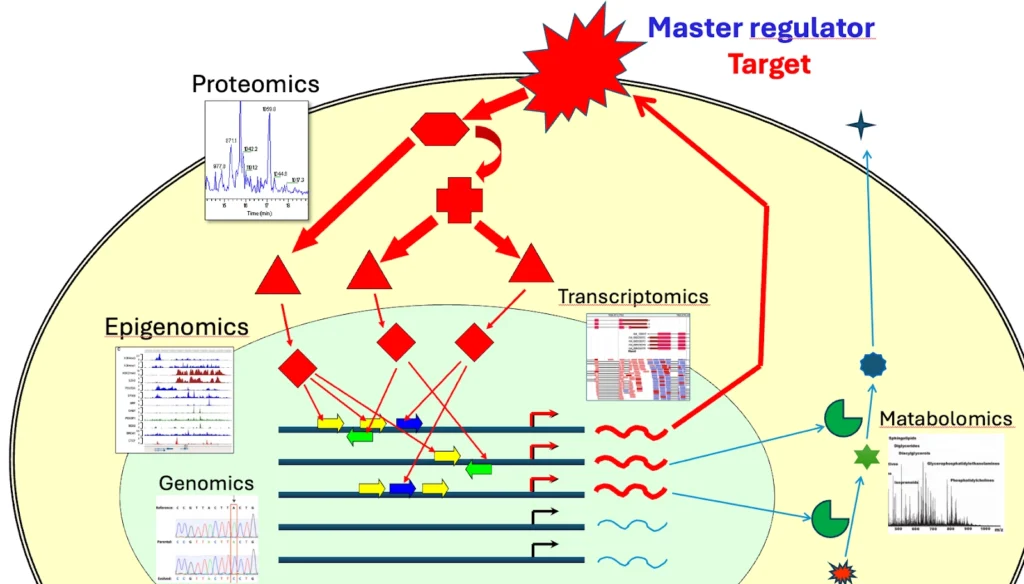
Drug repurposing:
Genome Enhancer can screen for existing FDA-approved drugs that interact with the disease-specific targets, identifying candidates for repurposing. Through pathway and network analysis, the tool evaluates the potential efficacy of repurposed drugs by assessing their ability to modulate critical disease pathways.
Fully automatic:
Genome Enhancer offers a fully automatic, one-click solution that revolutionizes the way omics data is analyzed and interpreted. With its user-friendly interface and cutting-edge algorithms, it eliminates the need for manual data processing, allowing researchers to focus on their discoveries. The platform’s graphical experiment design feature simplifies the setup of complex analyses, ensuring that every step is optimized and scientifically robust. This streamlined process empowers users to extract meaningful insights with minimal effort, making advanced bioinformatics accessible to both experts and newcomers alike.
Detailed report:
Genome Enhancer delivers a comprehensive and detailed report that includes all the essential elements for publication-ready research. From enriched pathways and gene networks to master regulator identification and statistical validations, every aspect of the analysis is meticulously documented. The report is not only informative but also formatted to meet the high standards of scientific journals, saving researchers significant time and effort in manuscript preparation. With Genome Enhancer, you can seamlessly transition from data analysis to impactful publication.
Only three steps to launch the analysis
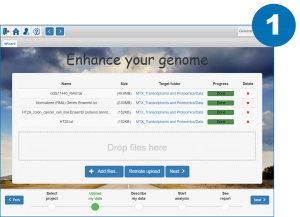
Upload your data to the server and specify the import options (data type)
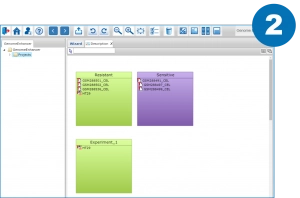
Split your data by the conditions you want to compare
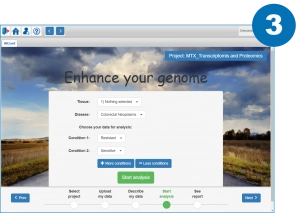
Launch the analysis by specifying the conditions to be compared and the disease and tissue types (optional)
The analysis report will be ready shortly. Depending on your input data, it will include lists of differentially expressed or mutated genes; transcription factors, regulating those genes; reconstructed signaling network of the studied pathological process; potential drug targets and corresponding known drugs and repurposing drugs, which may be effective in the studied case, as well as further cheminformatically predicted drug-like compounds. The report also contains description of analysis methods used and the references.
Acceptable input data formatsGenome Enhancer works with genomics, transcriptomics, epigenomics, proteomics and metabolomics input data types of the following formats:
Transcriptomics (RNA-seq, microarrays)
*.txt, *.csv, *.xls (table with gene identifiers)
*.CEL (affymetrix)
*.txt (special agilent format)
*.txt (special illumina format)
*.fastq
Epigenomics (ChIP-seq)
*.fastq
*.bam (hg38 only)
*.bed (hg38 only)
*.txt (table with illumina methylation probe ids, cg*)
Genomics
*.vcf
*.txt, *.csv, *.xls (table data with SNP identifiers, rs*), *.tsv
*.fastq
Proteomics
*.txt, *.csv, *.xls (table with protein identifiers)
Metabolomics
*.txt, *.csv, *.xls (table with the list of metabolites from chebi database, e.g. CHEBI:57316)
Files of one data format can be uploaded in a .zip archive
Report examples
You can view various analysis report examples generated by Genome Enhancer on the basis of different omics input data types and various origins of the studied pathologies:
- Colorectal Cancer (Personalized patient data) — Genomics, VCF
- MTB (Molecular Tumor Board) report example for colorectal cancer patient — Genomics, VCF
- Esophageal Squamous Cell Carcinoma (GSE32424) — Transcriptomics, FASTQ
- IFN-alpha induction (GSE31193) — Transcriptomics, LogFC Table
- Lung cancer, treatment by TGF (ST000010) — Metabolomics, Table
- Osteosarcoma, neoplasm metastasis (GSE66789) — Transcriptome + Proteome, RNA-seq + Mass-spec proteomics
- Ovarian cancer, cisplatin-resistance (GSE15709) — Transcriptomics + Epigenomics, CEL + BED
- SNP associated with Diabetes Mellitus — Genomics, SNP list
- Parkinson disease, induced a-Syn expression in SH-SY5Y cells (GSE145804) — Transcriptomics, LogFC Table
- Non-Small Cell Lung Carcinoma (NCI-H1975) — Genomics, VCF
- MTB (Molecular Tumor Board) report example for non-small cell lung carcinoma (NCI-H1975) — Genomics, VCF
- Hypertension (GSE157131) — Epigenomics, cg lists
TRANSFAC DOWNLOAD
Download TRANSFAC and do whatever you like
Introduction
TRANSFAC flat file download (including the databases TRANSCompel® and TRANSProTM) contains eukaryotic transcription factors (and miRNAs), their experimentally determined genomic binding sites and consensus DNA-binding motifs (PWMs), as well as data on combinatorial gene regulation and factor-factor interaction. Promoters, enhancers and silencers annotated with transcription factor ChIP-Seq, DNase hyper-sensitivity and histone methylated intervals from the ENCODE project and from other sources complement the manually curated binding site data.
Key features
- Intended for Bioinformaticians
- No installation is needed – just download and unzip archives
- Data files are provided in DAT and JSON formats
- Promoters are provided in the DAT and GTF formats
- Direct data access without user interface: data extraction is possible via Perl scripts or other programs written by the user
- Java-based tools for TFBS search (Match Library) are accessible via a command line
- For use with customer tools and incorporation into user-specific pipelines
What you will get
- Based on the positional weight matrices (PWMs) transcription factor binding sites can be predicted in regulatory regions.
- In the TRANSFAC® flat file download, the tools of the MatchTM Library can be used on command line or the PWMs can be used with tools of the user.
YOUR BENEFITS USING TRANSFAC 2.0
MOTIFS AND PREDICTION OF TF-BINDING SITES
Use the most comprehensive library of known eukaryotic transcription factor binding motifs
TRANSFAC systematically collects all available TF-binding motifs in the form of Positional Weight Matrices (PWMs) from scientific literature and repositories, as well as PWMs constructed by the TRANSFAC team on the basis of experimentally verified TF binding sites. Currently TRANSFAC provides more than 10,000 PWMs for various eukaryotic taxonomic groups. Our goal is to provide the most comprehensive resource of TF binding motifs for researchers world-wide
Identify common motifs in a set of target DNA sequences
Determine common motifs and compare these de-novo motifs to known transcription factor DNA binding site consensus sequences present in the TRANSFAC database
Detect genomic variants affecting TF-binding sites
Analyze mutations from your NGS data in regulatory regions for their potential negative or positive effect on transcription factor binding
Predict TF-binding sites in eukaryotic DNA sequences
Our tools predict transcription factor (TF) binding sites and composite regulatory regions using Machine Learning (ML) and Artificial Intelligence (AI)
PROMOTERS AND ENHANCERS
The unrivaled resource for studying promoters and enhancers
Due to its comprehensive data on transcription factors and their binding sites, tools for motif analysis, support for cross-species comparisons and functional annotations, TRANSFAC is an indispensable resource for studying promoters and enhancers
Find known transcriptional regulators for your gene(s) of interest
Search for factor-gene interactions in TRANSFAC, the largest collection of published experimentally proven transcription factor binding sites
Explore factor-factor interactions and composite elements
Complement the unparalleled collection of factor-gene interactions with factor-factor interactions and synergistic and antagonistic composite elements
Predict target genes
Find target genes for a transcription factor of interest by studying from single gene promoters to whole genomes
Analyze genes for tissue- and GO-specific transcription factors
Select tissue- / cell type- / induction-specific transcription factors for genes from human and model organisms
PATHWAYS AND MASTER REGULATORS
Identify pathways up- and down-stream of a gene (set)
Explore activation patterns of genes in tissues and cells of your interest and build complex interaction networks based on individual reactions with experimental details, protein-protein interactions (PPIs) and post-translational modifications (PTMs) in TRANSFAC PATHWAYS
Apply integrated network analysis and visualization
Profit from the combined approach towards causative gene regulation studies. Explore activation patterns of genes in tissues and cells of your interest and build complex interaction networks with identified master regulators
Map gene sets on pathways
Draw insights on biological function of your gene set by mapping them on pathways
Customize regulatory and metabolic networks
Build networks based on more than one million reactions extracted from original scientific literature and evaluated by experts.
MULTI-OMICS
Easily process and integrate all your omics data with TRANSFAC PATHWAYS / DISEASES
Preprocess, functionally explore, and unite various omics data (genomics, transcriptomics, metabolomics, proteomics and epigenomics) in a fully automized pipeline and get a combined and integrated report
Find common functional properties in a set of (co-regulated) genes
Map your data on various ontologies and identify overrepresented functional assignments in your gene set
Compare and functionally align your data
Observe how your omics data sets (genomics, transcriptomics, proteomics, epigenomics or metabolomics) correlate between each other
Utilize upstream analysis
Benefit from our unique upstream analysis approach combining promoter and pathway analysis to identify transcription factors and upstream master regulators (as potential drug targets) which can explain expression changes of your DEGs (or other changes in gene or protein signatures)
BIOMARKERS, DRUGS AND COMPOUNDS
Discover disease molecular mechanisms
Make use of the vast amount of gene-disease and gene-drug assignments and identify novel biomarkers and drug targets
Reconstruct disease molecular mechanism
Understand the drug’s mechanism of action (MoA) based on the collected omics data
Trace back the activated pathways
Detect disease master regulators, responsible for governing the pathology development processes, and therapeutic targets
PRECISION MEDICINE
Employ personalized medicine with TRANSFAC DISEASES
With our fully automated pipeline for patient’s multi-omics data analysis TRANSFAC DISEASES generates a comprehensive report about the personalized drug targets identified for a certain patient, or a group of patients, and the potentially effective drugs. Application examples include cancer, neurodegenerative diseases, infectious diseases, diabetes, metabolic diseases and hypertension
Develop a personalized therapy
Identify individual drug targets and corresponding treatments based on the pathology molecular mechanism reconstructed on omics data collected from a particular patient
Repurpose drugs
Explore how known drug targets can be activated in various pathologies. Check out the possible off-label usage of treatments and identify prospective drug combinations for better patient outcomes
Find new drug candidates
Identify novel drug targets and find prospective drug-like compounds potentially acting on them by using integrated promoter, pathway and cheminformatics analysis
GENERAL
Inbuilt workflows
Make use of over 200 pre-compiled workflows
Customizable pipelines
Construct your own dedicated analysis pipeline with visual programming
Integrated Genome Browser
Get your result in tabular format as well as in the integrated genome browser
Application Programming Interface (API)
Use Java-based API, R-based API or Jupiter notebook
Pathway/Network visualization
Visualize canonical pathways and analysis-dependent networks
Comprehensive analysis reports
Profit from automatically generated analysis reports including network visualizations, functional annotation diagrams and more
WHAT MAKES TRANSFAC 2.0 DIFFERENT FROM OTHER TOOLS?
- Most comprehensive database on gene regulation
TRANSFAC stands as the pioneering and most comprehensive database on eukaryotic transcription factors (TFs), their genomic binding sites (TFBS), and DNA binding profiles (PWMs).
- 35 years of curation and maintenance
Once established over 35 years ago, TRANSFAC has been diligently maintained and manually curated ever since.
- The biggest collection of experimentally proven functional TF binding sites
TRANSFAC 2.0 contains the biggest collection of experimentally proven TF binding sites that regulate expression of genes in genomes of eukaryotic organisms curated from original publications and documented with detailed information about tissue, cell types, TF source and quality of experimental evidence.
- The largest library of Positional Weight Matrices (PWMs)
TRANSFAC 2.0 contains over 10,000 DNA binding patterns in the format of positional weight matrices (PWMs) for animals, plants and fungi. PWMs are built based on experimentally proven TF binding sites, curated from original scientific publications and integrated from other databases.
- Signal transduction network of more than 1,200,000 reactions
TFs are connected to a network of more than 1,200,000 of signal transduction and metabolic reactions extracted from original scientific literature and evaluated by experts. Over 1500 canonical pathways are described based on these reactions.
- Unique algorithm to find master-regulators
Master-regulators are discovered by the “upstream analysis” that uniquely integrates promoter and network analysis using graph search and genetic algorithms.
- Biggest collection of more than 140,000 disease biomarkers
Manually curated collection of more than 140,000 gene to disease associations as correlative, causal and disease mechanisms biomarkers and drug targets.
- Reconstruction of disease molecular mechanisms based on the upstream analysis
Combining upstream analysis approach and disease and pathway information allows to reconstruct disease mechanisms and find novel drug targets.
- Over 300 powerful tools and pipelines to study gene regulation
TRANSFAC 2.0 provides a platform of multiple web tools and ready pipelines for analysis of NGS, RNA-seq, ChIP-seq, ATAC-seq, CUT&RUN and other types of genomics, transcriptomics, epigenomics, proteomics and metabolomics data. No cumbersome installation or special bioinformatics skills are needed.
- Robust AI algorithms for promoter and enhancer analysis
Integration of powerful tools for scanning genomes for TF binding sites and for discovering site enrichment and site combinatorial modules using AI, such as genetic algorithms, and machine learning.
- Automatic multi-omics discovery pipeline “Genome Enhancer”
Genome Enhancer provides a fully automated pipeline, including report, for patient omics data analysis, which identifies prospective drug targets and corresponding treatments by reconstructing the molecular mechanism of the studied pathology.
TRANSFAC versus JASPAR
Feature 9003241321086270_89d334-45> |
TRANSFAC 9003241321086270_4a90ed-5a> |
JASPAR 9003241321086270_f50187-43> |
---|---|---|
Database statistics 9003241321086270_e75479-23> |
Factors – 48,258 DNA Sites – 50,892 Factor-DNA Site Links – 68,900 Genes – 102,973 Matrices – 10,706 References – 45,130 9003241321086270_5f34f4-ee> |
– No DNA Sites -2,000 profiles (Matrices) in JASPAR core (2024 release) 9003241321086270_9940af-1f> |
Database statistics (miRNA) 9003241321086270_b3a718-ea> |
miRNAs – 1,772 mRNA Sites- 67,703 miRNA-mRNA Site Links – 74,553 9003241321086270_89f830-b7> |
No miRNA data 9003241321086270_828793-10> |
Database statistics |
Distinct transcription factors in Chip-seq experiment : 1,171 TF-TG associations : 15,639,406 ChIP TFBS : 95,867,624 9003241321086270_2ddfb6-29> |
No Chip-seq data. 9003241321086270_06526f-2c> |
Data Depth 9003241321086270_6f32b4-37> |
Genome annotation of experimentally validated TF binding sites Genome annotation of enhancers, genome conserved regions. 9003241321086270_d6b83b-c5> |
Limited to binding motifs 9003241321086270_ec152c-1a> |
Data Quality 9003241321086270_c64096-9c> |
Combines public and proprietary datasets, enhancing dataset completeness. 9003241321086270_437011-d0> |
Restricted only to open-access data. 9003241321086270_23d605-39> |
Data Integration 9003241321086270_be11b3-12> |
Links TF binding site data with additional omics data, including epigenetic modifications and expression profiles. Supports multi-layered analyses that combine DNA-protein interactions and gene expression. 9003241321086270_64e843-72> |
Focuses on TF motifs and provides limited integration with other datasets. 9003241321086270_030569-e7> |
Integrated Pathway Analysis 9003241321086270_1a3cef-83> |
Supports integrated promoter and pathway analysis allowing to identify Master Regulators of the studied processes, which in their turn can serve as prospective disease mechanism-based biomarkers and drug targets 9003241321086270_8458bd-65> |
Limited exclusively to promoter analysis with no further pathway analysis extensions supported 9003241321086270_43b733-3b> |
Additional tools 9003241321086270_711ae6-48> |
Offers tools like MATCH™ for TFBS prediction and analysis., Click and Run pipelines integrating TRANSFAC for identifying enriched binding sites, composite modules, combinatorial analysis 9003241321086270_cd2c62-63> |
No own tools. Linked to third-party tools for motif scanning and sequence analysis 9003241321086270_224634-aa> |
AI-based extensions 9003241321086270_b43700-8b> |
Includes AI and ML based methods for prediction of TFBS combinations, including construction of composite modules based on a genetic 9003241321086270_a41bb9-cb> |
Limited to standard approached towards motif scanning and sequence analysis 9003241321086270_027817-b3> |
Clinical Relevance 9003241321086270_fd6b74-62> |
Annotated for disease-related transcription factors and binding sites. In addition to biomarker info, includes annotations for drug-disease-clinical trials relations 9003241321086270_bf3322-d0> |
Minimal disease annotations 9003241321086270_f28127-18> |
Species 9003241321086270_75eb97-5c> |
Includes data on multiple species of vertebrates, nematodes, yeast, insects, plants. TRANSFAC is integrated with geneXplain platform and provides flexibility to integrate new custom genomes and identify transcription factor binding sites 9003241321086270_d865d7-a8> |
Includes TF binding motifs for six organism classes. Integration of new custom genomes is not provided 9003241321086270_35e2ee-23> |
Customer Support 9003241321086270_5d6a03-f4> |
Regular updates, Prompt customer support with technical assistance by experts in the industry 9003241321086270_f39b09-0c> |
Open-source platform, assistance through documentation 9003241321086270_f9d0a5-18> |
Accessibility 9003241321086270_69c93c-fd> |
Flexible, affordable and customized packages available to access total TRANSFAC functionality 9003241321086270_400545-cf> |
Freely accessible for academic and non-commercial research 9003241321086270_b3b9ba-7f> |
Selection of articles reporting about HumanPSD applications:
- Kawashima Y., Nagai H., Konno R., Ishikawa M., Nakajima D., Sato H., Nakamura R., Furuyashiki T., Ohara O. (2022) Single-Shot 10K Proteome Approach: Over 10,000 Protein Identifications by Data-Independent Acquisition-Based Single-Shot Proteomics with Ion Mobility Spectrometry. J Proteome Res. 21(6), 1418–1427. Link
- Lim, J. S., Ibaseta, A., Fischer, M. M., Cancilla, B., O’Young, G., Cristea, S., Luca, V. C., Yang, D., Jahchan, N. S., Hamard, C., Antoine, M., Wislez, M., Kong, C., Cain, J., Liu, Y. W., Kapoun, A. M., Garcia, K. C., Hoey, T., Murriel, C. L., & Sage, J. (2017). Intratumoural heterogeneity generated by Notch signalling promotes small-cell lung cancer. Nature, 545(7654), 360–364. Link
- Reales‐Calderón, J. A., Aguilera‐Montilla, N., Corbí, Á. L., Molero, G., & Gil, C. (2014). Proteomic characterization of human proinflammatory M1 and anti‐inflammatory M2 macrophages and their response to Candida albicans. Proteomics, 14(12), 1503-1518. Link
- Martínez‐Solano, L., Nombela, C., Molero, G., & Gil, C. (2006). Differential protein expression of murine macrophages upon interaction with Candida albicans. Proteomics, 6(S1), S133-S144. Link
Publications
Selection of publications authored by the geneXplain team:
- Kisakol, B., Matveeva, A., Salvucci, M., Kel, A., McDonough, E., Ginty, F., Longley, D., Prehn, J. (2024) Identification of unique rectal cancer-specific subtypes. Br J Cancer. DOI https://doi.org/10.1038/s41416-024-02656-0. Link
- Kolpakov, F., Akberdin, I., Kiselev, I., Kolmykov, S., Kondrakhin, Y., Kulyashov, M., Kutumova, E., Pintus, S., Ryabova, A., Sharipov, R., Yevshin, I., Zhatchenko, S., & Kel, A. (2022). BioUML-towards a universal research platform. Nucleic Acids Res. 50(W1),W124–31. Link
- Orekhov A.N., Sukhorukov V.N., Nikiforov N.G., Kubekina M.V., Sobenin I.A., Foxx K.K., Pintus S., Stegmaier P., Stelmashenko D., Kel A., Poznyak A.V., Wu W.K., Kasianov A.S., Makeev V.Y., Manabe I., Oishi Y. (2020) Signaling Pathways Potentially Responsible for Foam Cell Formation: Cholesterol Accumulation or Inflammatory Response-What is First? Int J Mol Sci. 21(8),2716. Link
- Kel A., Boyarskikh U., Stegmaier P., Leskov L.S., Sokolov A.V., Yevshin I., Mandrik N., Stelmashenko D., Koschmann J., Kel-Margoulis O., Krull M., Martínez-Cardús A., Moran S., Esteller M., Kolpakov F., Filipenko M., Wingender E. (2019) Walking pathways with positive feedback loops reveal DNA methylation biomarkers of colorectal cancer. BMC Bioinformatics. 20(Suppl 4),119. Link
- Boyarskikh, U., Pintus, S., Mandrik, N., Stelmashenko, D., Kiselev, I., Evshin, I., Sharipov, R., Stegmaier, P., Kolpakov, F., Filipenko, M., Kel, A. (2018) Computational master-regulator search reveals mTOR and PI3K pathways responsible for low sensitivity of NCI-H292 and A427 lung cancer cell lines to cytotoxic action of p53 activator Nutlin-3. BMC Med. Genomics 11(Suppl 1), 12. Link
Kel, A.E., Stegmaier, P., Valeev, T., Koschmann, J., Poroikov, V., Kel-Margoulis, O.V. and Wingender, E. (2016) Multi-omics “upstream analysis” of regulatory genomic regions helps identifying targets against methotrexate resistance of colon cancer. EuPA Open Proteomics 13, 1-13. Link