Genome Enhancer features
Disease mechanism:
Genome Enhancer applies AI algorithms such as Genetic Algorithm and complex graph analysis algorithms to discover disease molecular mechanisms and to identify potential drug targets.
The analysis can be done in the context of over 3,900 different human diseases, including complex diseases such as cancer, cardio-vascular, auto-immune, neurodegenerative diseases as well as multiple genetic and rare diseases.
Reconstructed disease molecular mechanism of Glioblastoma tumors by comparing long-survival versus short-survival patient transcriptomics (RNA-seq data)
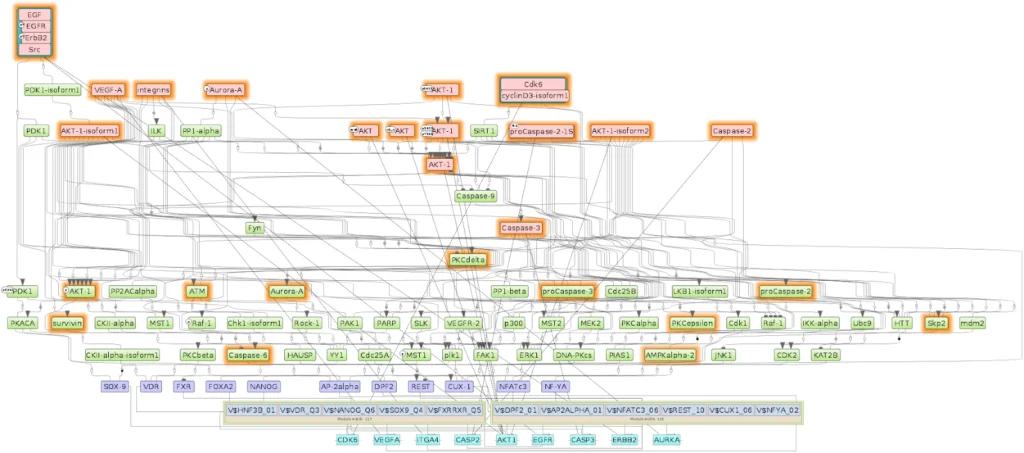
Multi-omics integration:
Genome Enhancer provides flexible integration of all five “-omics” data types: Transcriptomics, Genomics, Epigenomics, Proteomics, Metabolomics. Any combinations as well as individual omics data can be combined in one analysis run.
The omics integration is done following the principles of organisation of molecular-biological and biochemical systems in eukaryotic cells. The Upstream Analysis integrates promoter and pathway analysis, to identify potential drug targets of the studied pathology. Transcriptomics data help to find differentially expressed genes (DEGs); Metabolomics help to reveal which of the DEGs are most critical for metabolome changes in the studied pathology; Epigenomic data help to identify most regulatory active genomic regions for searching for TFBS enrichment; Genomic data help to reveal TF binding sites affected by regulatory mutations; Proteomics data help to strengthen the master regulator search.
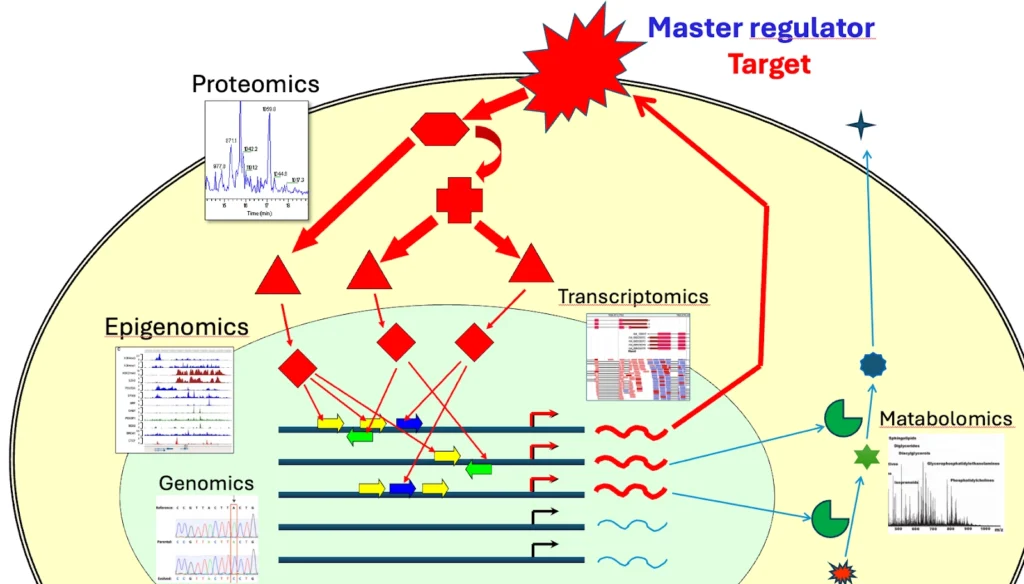
Acceptable input data formats
Genome Enhancer works with genomics, transcriptomics, epigenomics, proteomics and metabolomics input data types of the following formats:
Transcriptomics (RNA-seq, microarrays)
*.txt, *.csv, *.xls (table with gene identifiers)
*.CEL (affymetrix)
*.txt (special agilent format)
*.txt (special illumina format)
*.fastq
Epigenomics (ChIP-seq)
*.fastq
*.bam (hg38 only)
*.bed (hg38 only)
*.txt (table with illumina methylation probe ids, cg*)
Genomics
*.vcf
*.txt, *.csv, *.xls (table data with SNP identifiers, rs*), *.tsv
*.fastq
Proteomics
*.txt, *.csv, *.xls (table with protein identifiers)
Metabolomics
*.txt, *.csv, *.xls (table with the list of metabolites from chebi database, e.g. CHEBI:57316)
Files of one data format can be uploaded in a .zip archive
Drug repurposing:
Genome Enhancer can screen for existing FDA-approved drugs that interact with the disease-specific targets, identifying candidates for repurposing
Through pathway and network analysis, the tool evaluates the potential efficacy of repurposed drugs by assessing their ability to modulate critical disease pathways.
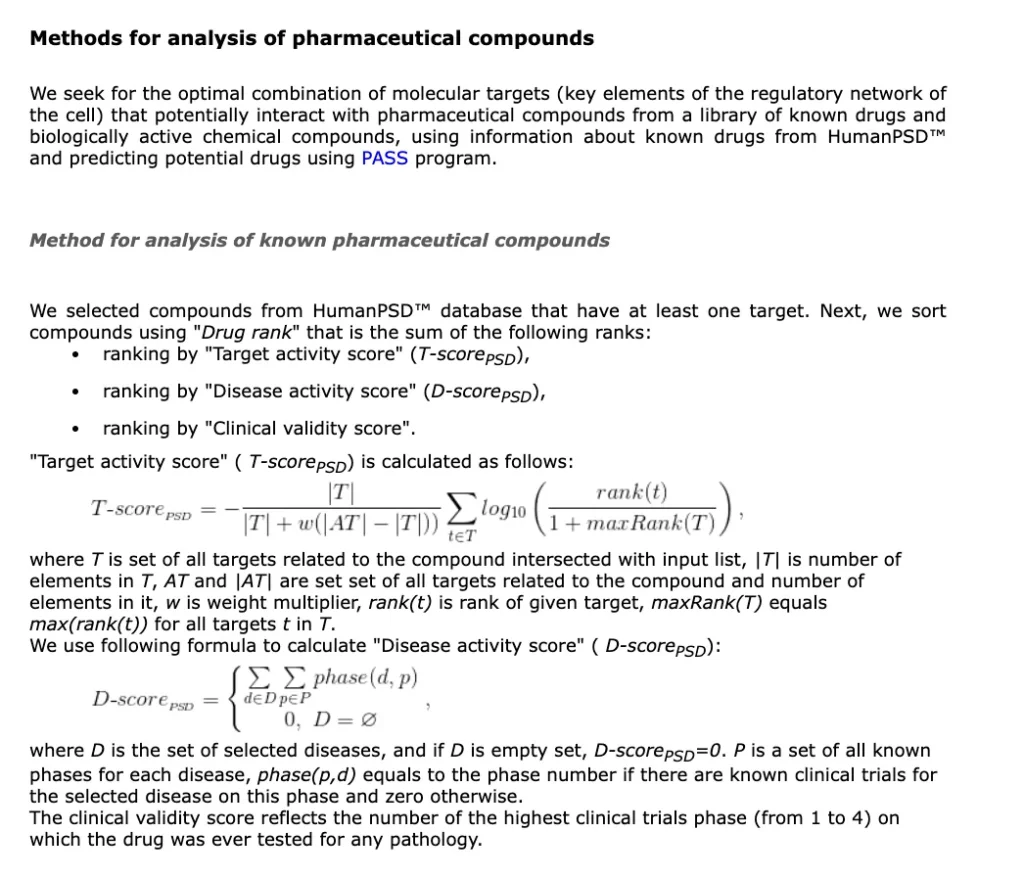
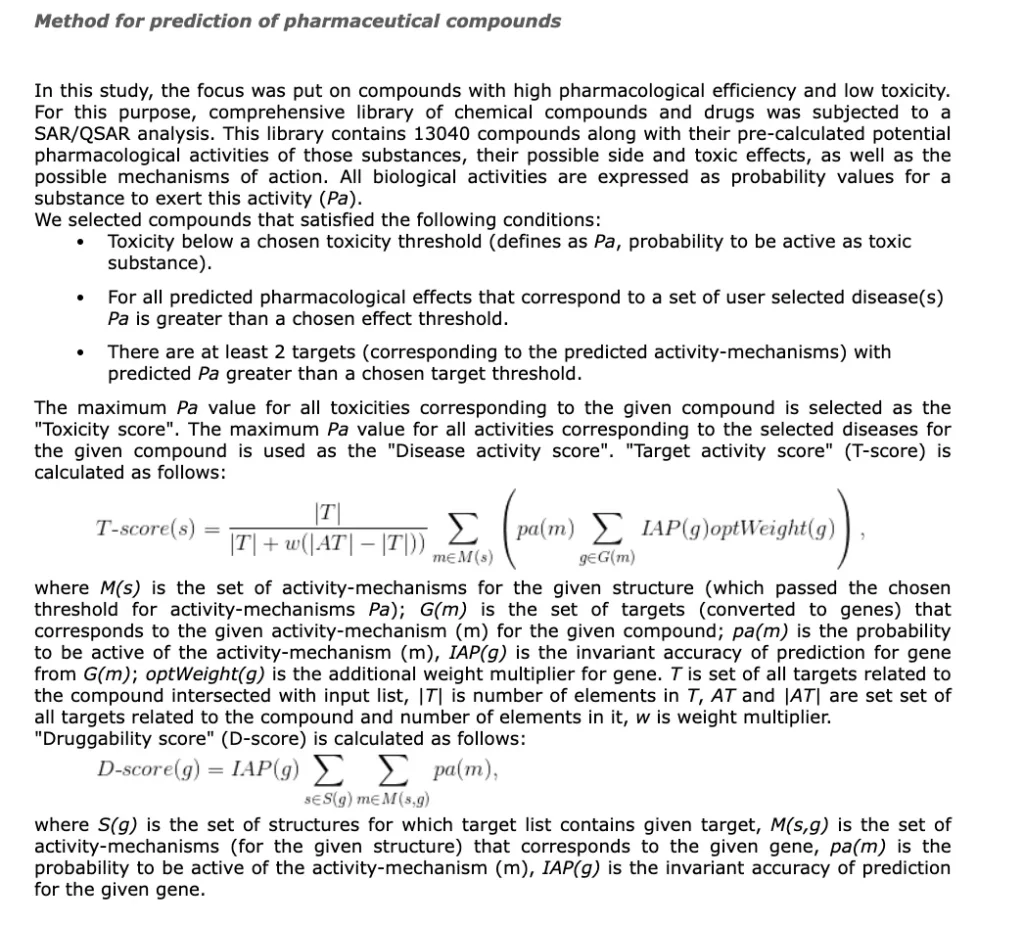
As a result Genome Enhancer computes repurposing drugs as below:
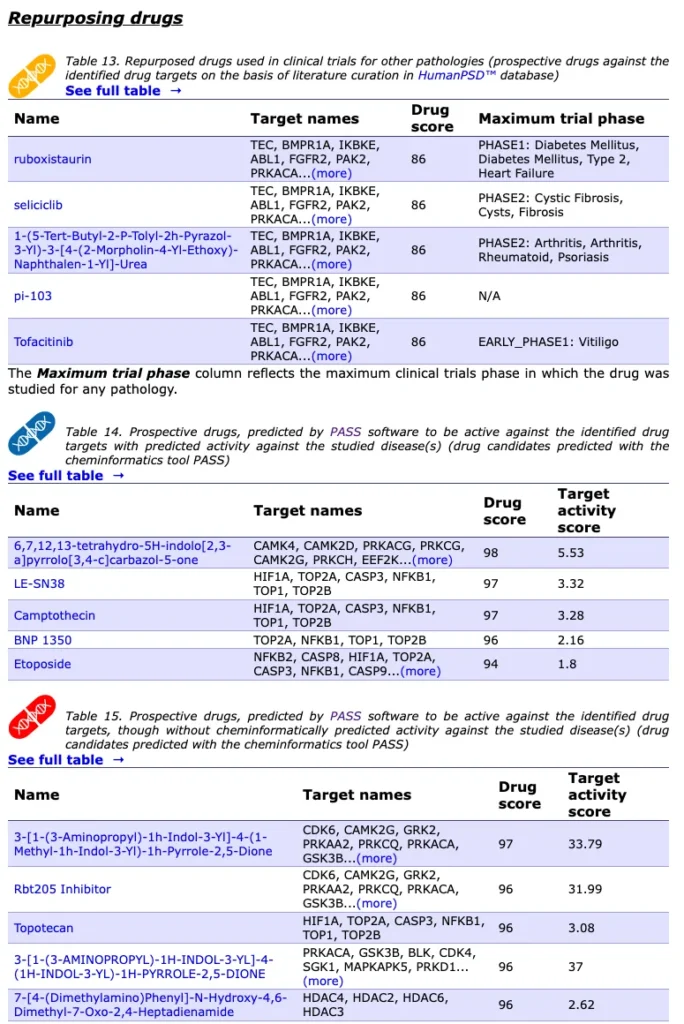
Detailed report:
Genome Enhancer delivers a comprehensive and detailed report that includes all the essential elements for publication-ready research.
Publication-ready research
From enriched pathways and gene networks to master regulator identification and statistical validations, every aspect of the analysis is meticulously documented. The report is not only informative but also formatted to meet the high standards of scientific journals, saving researchers significant time and effort in manuscript preparation. With Genome Enhancer, you can seamlessly transition from data analysis to impactful publication.
Only three steps to launch the analysis
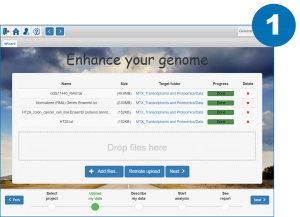
Upload your data to the server and specify the import options (data type)
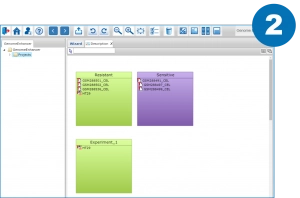
Split your data by the conditions you want to compare
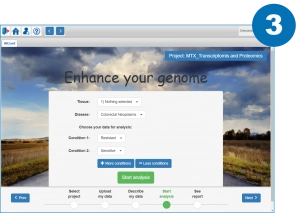
Launch the analysis by specifying the conditions to be compared and the disease and tissue types (optional)
The analysis report will be ready shortly. Depending on your input data, it will include lists of differentially expressed or mutated genes; transcription factors, regulating those genes; reconstructed signaling network of the studied pathological process; potential drug targets and corresponding known drugs and repurposing drugs, which may be effective in the studied case, as well as further cheminformatically predicted drug-like compounds. The report also contains description of analysis methods used and the references