GUSAR
Create quantitative models on structure-activity (structure-property) relationships.
Run your compounds on pre-computed quantitative models
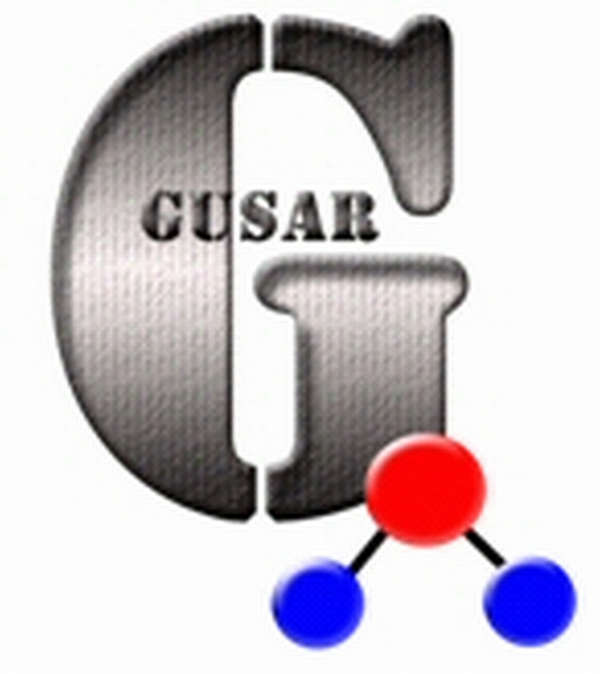
Introduction
GUSAR is a tool to create models on quantitative structure-activity relationships. The acronym stands for “General Unrestricted Structure-Activity Relationships”.The input of the program is your training set of chemical structures and quantitative data on biological activities. The output is a reliable quantitative SAR/SPR (Structure Activity and Property Relationship) model.
GUSAR user interface
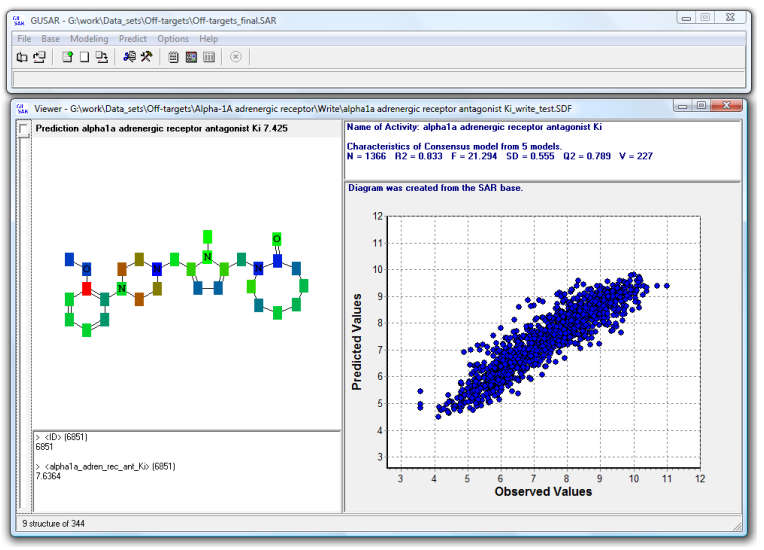
Quantitative prediction of the effect of a chemical compound.
Watch this video to gain an impression about GUSAR’s look-and-feel:
GUSAR is a tool for analysis of quantitative and qualitative structure-activity/structure-property relationships ((Q)SAR/(Q)SPR) on the basis of the structural formulas of the compounds and data on their activity/property, and for prediction of activity/property for new compounds. The acronym stands for “General Unrestricted Structure-Activity Relationships”. GUSAR can be easily applied to different routine (Q)SAR tasks, for building many models, and for prediction by these models of the different quantitative/qualitative values simultaneously.
The GUSAR software provides the following functions:
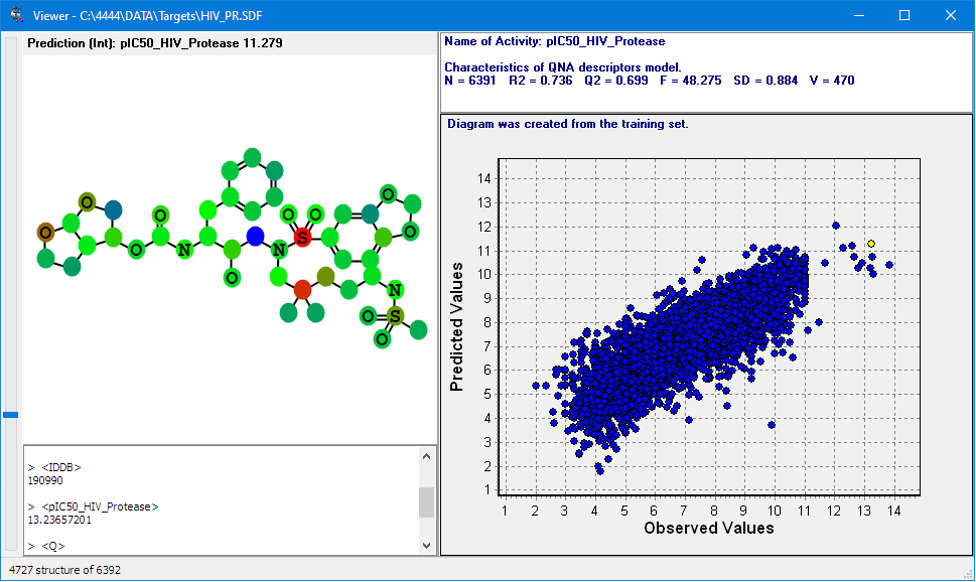
GUSAR features and applications
Last release features:
Automatic, simplified creation of (Q)SAR models
Enhanced choice of variables:
Improved algorithm of model building and prediction:
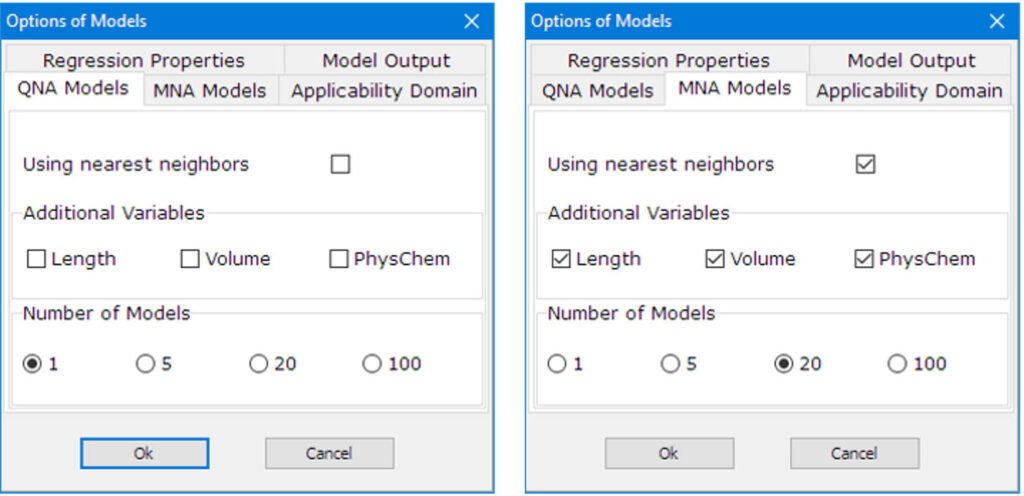
GUSAR algorithm
The core of GUSAR consists of a unique algorithm of self-consistent regression that allows to select the best set of descriptors for a robust and reliable QSAR model.
Chemical structures are represented by MNA (Multilevel Neighborhood of Atoms) or QNA (Quantitative Neighbourhoods of Atoms) descriptors and biological activity descriptors that are based on the PASS prediction results for more than 8000 biological activities. QNA descriptors easily reflect the nature of intermolecular interactions. Models developed using biological activity descriptors enable to reveal key mechanisms of action of complex biological effects. MNA and QNA descriptors are used to calculate several variables, such as topological length and volume or lipophilicity of a molecule. For further details, see Filimonov et al. (2009), Zakharov et al. (2012), Zakharov et al. (2016).
GUSAR competitive advantages
The program allows creating individual (Q)SAR models and their sets, presented in the form of consensus as well.
It can be used for the creation of (Q)SAR models for the prediction of properties of organic compounds belonging to both homogeneous and heterogeneous chemical classes.
It is based on unique and innovative atom centric descriptors which are called Quantitative and Multilevel Neighborhoods of Atoms (QNA and MNA) descriptors.
It uses modern and robust machine learning approaches: self-consistent regression and radial basis functions for automatic creation of (Q)SAR models.
Along with prediction results the end-user can get an evaluation of applicability domain of the model.
Visualization of contributions of atoms into predicted value of the activity allows identifying the atoms and molecular fragments that make a positive and a negative contribution to the activity.
User-friendly interface, fast speed of the (Q)SAR models creation and prediction of the test compounds as well.
GUSAR in comparison
In comparison with a number of 3D and 2D QSAR methods, the predictivity of GUSAR was superior to that of most other QSAR methods both on heterogeneous and on homogeneous data sets.
(Filimonov et al., 2009)
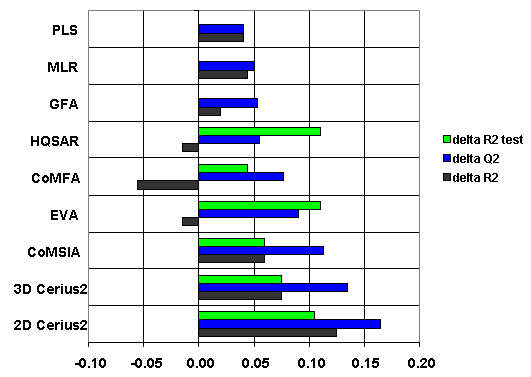
Comparison of different QSAR approaches; shown is the performance of GUSAR relative to other methods.
Precomputed GUSAR models
The GUSAR software optionally includes ready-trained GUSAR models (SAR bases) for predicting certain biological activities. These are SAR bases that can be used with the GUSAR program for predictions on acute rat toxicity, acute mouse toxicity or antitargets (off-targets).
The acute rat or mouse toxicity SAR bases can be used for in silico prediction of LD50 values for rats or mouse with four types of administration.
A quantitative prediction of antitarget interaction for chemical compounds can be done with the other SAR base. The QSAR models for the set of 32 activities (using IC50, Ki or Kact values) includes data on about 4,000 chemical compounds interacting with 18 antitarget proteins (13 receptors, 2 enzymes and 3 transporters).
Acute Rat Toxicity
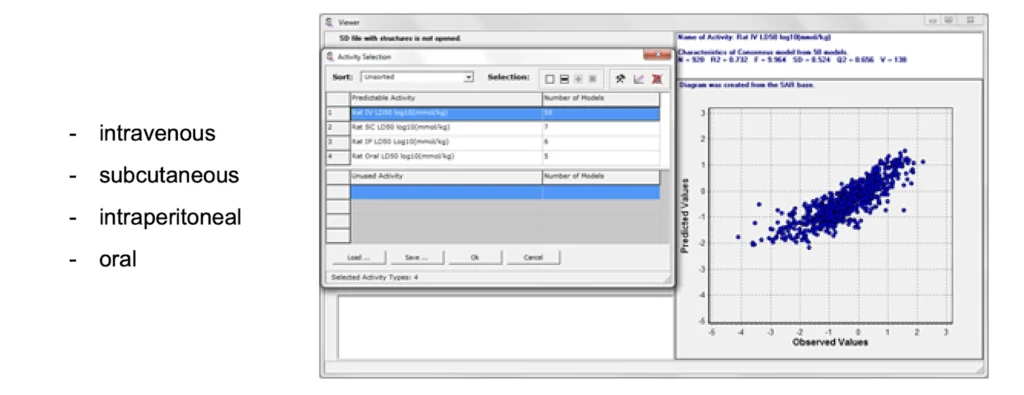
GUSAR publications
How to cite GUSAR
Filimonov DA, Zakharov AV, Lagunin AA, Poroikov VV. QNA-based ‘Star Track’ QSAR approach. SAR QSAR Environ Res. 2009 Oct;20(7-8):679-709. Link
Recent application of PASS and GUSAR, an example: Evaluation of inhibitors of Mycobacterium tuberculosis:
Pogodin PV, Salina EG, Semenov VV, Raihstat MM, Druzhilovskiy DS, Filimonov DA, Poroikov VV. Ligand-based virtual screening and biological evaluation of inhibitors of Mycobacterium tuberculosis H37Rv. SAR QSAR Environ Res. 2024 Jan;35(1):53-69. Link