PASS
Chemo-Informatics
Compute biological activity for a compound library
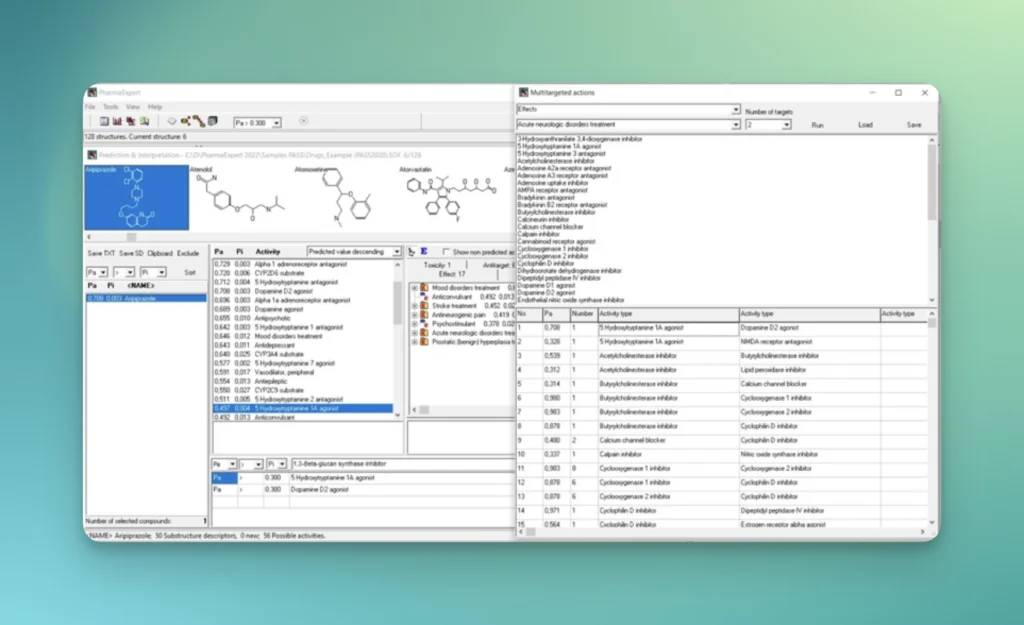
PASS with PharmaExpert
Compute biological activity for chemical compounds using SAR (structure-activity relationship) approach
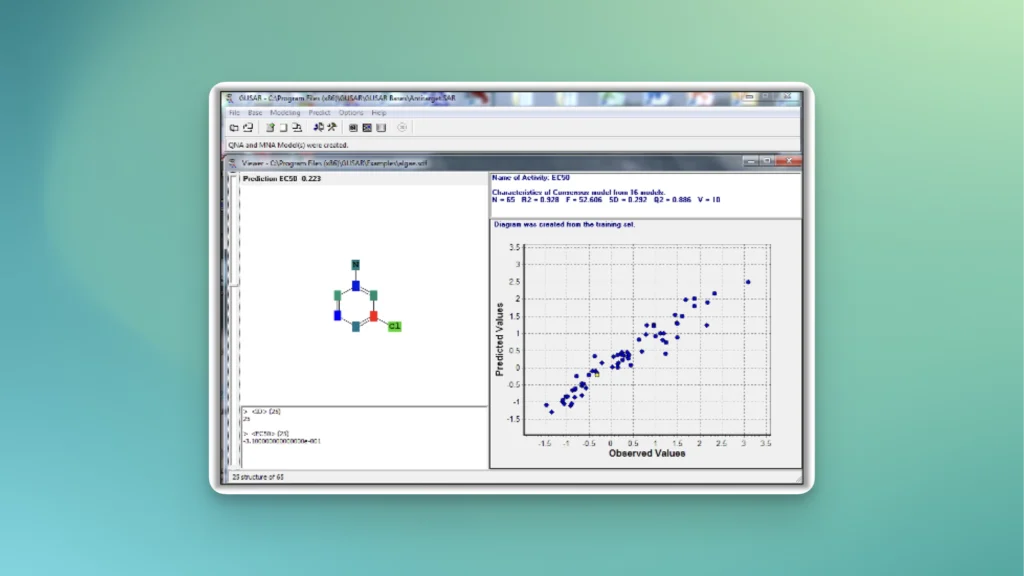
GUSAR
Create quantitative models on structure-activity (structure-property) relationships
PASS with PharmaExpert
Compute biological activity for chemical compounds using SAR (structure-activity relationship) approach
Introduction
The acronym PASS stands for Prediction of Activity Spectra for Substances. Using structural formula of a drug-like substance as an input, one obtains its estimated biological activity profile as an output. The predicted biological activity list includes the names of the probable activities with two probabilities: Pa – likelihood of belonging to the class of “Actives” and Pi – likelihood of belonging to the class of “Inactives”. By default, all activities with Pa>Pi are considered as probable; however, depending on the particular tasks, the user may choose any other cutoff for selecting the probable “Actives”.
PASS has been well accepted by the research community, and is now actively used in the field of medicinal chemistry, by both academic organizations and pharma companies. There are over 1,200 publications described PASS approach and its applications. Overview on some papers is provided here.
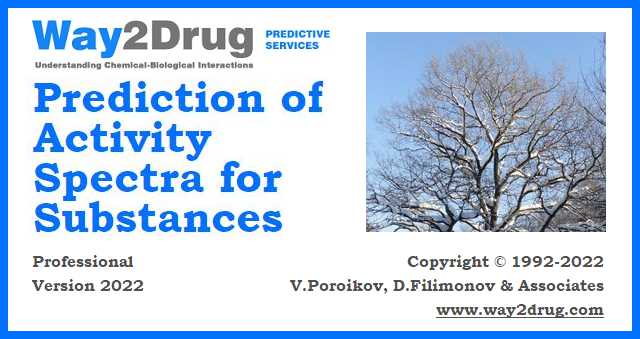

Activity prediction for a chemical substance by PASS
The slide show demonstrating the look-and-feel of PASS can be found here as well as on our Facebook site. A particularly useful tool to analyze and utilize PASS results further is PharmaExpert.
Current PASS statistics
PASS 2022 SAR Base is based on the information about structure-activity relationships of 1,614,066 substances; the total number of experimentally determined pairwise structure-activity records is 5,174,855. 8,565 biological activities can be predicted with average accuracy about 0.93; by default, 1,957 activities are predicted with average accuracy 0.97.
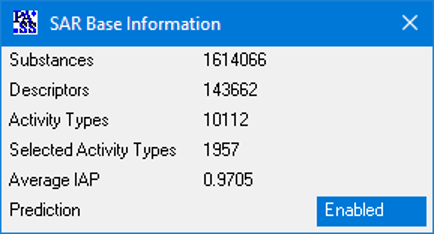
PASS features and applications
Main PASS features
PharmaExpert tool
PharmaExpert is an analyses tool to study the relationships between biological activities, drug-drug interactions and multiple targeting of chemical compounds and selects compounds that have a pre-defined biological activity. It helps answer a question like “How to select the most promising compounds among those known to interact with the selected protein?”
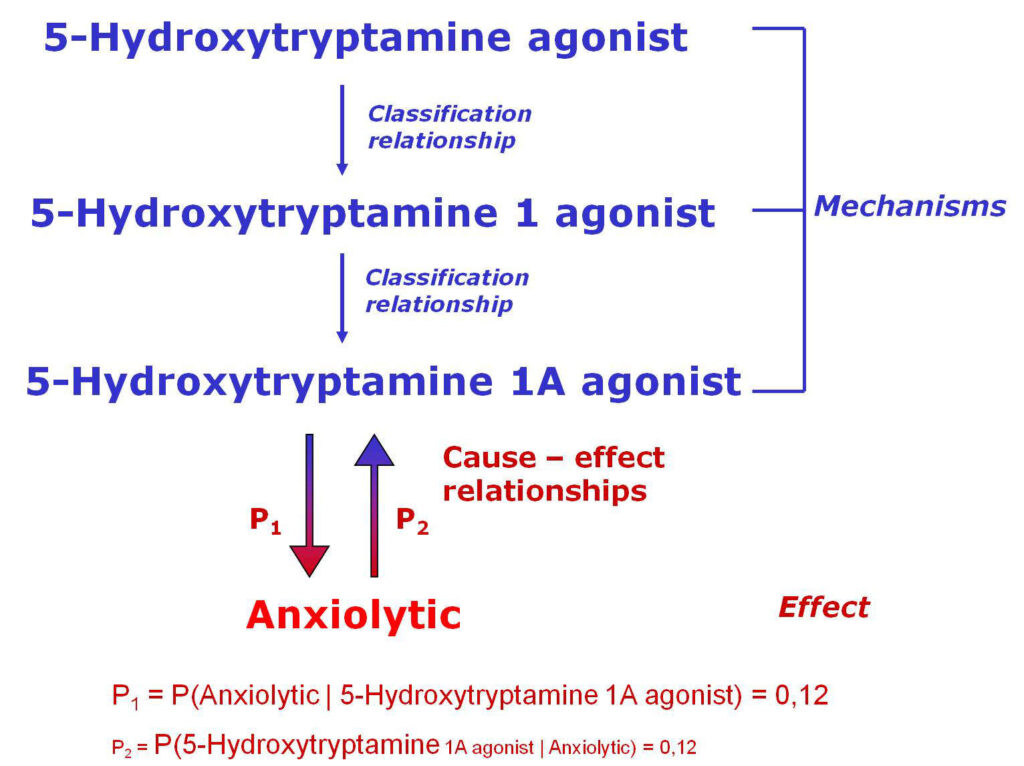
How PASS (Prediction of Activity Spectra for Substances) works?
PASS uses a structural formula of a drug-like substance as an input and computes its estimated biological activity profile (or spectrum) as an output. The predicted biological activity list includes the names of the probable activities with two probabilities: Pa – the likelihood of belonging to the class of “Actives” and Pi – the likelihood of belonging to the class of “Inactives”.
Key features of PASS 2022
PASS training set
The general PASS training set was corrected and extended; thus, PASS 2022 SAR Base includes 1,614,066 (1,368,353 in PASS 2020) drugs, drug-candidates, pharmaceutical agents and chemical probes, as well as compounds for which specific toxicity information is known.
Biological activities list
The entire activity list includes 10,112 terms describing biological activities (9,942 in PASS 2020). About two hundred novel biological activities were added including: Antiviral (Coronavirus), Antiviral (SARS coronavirus), 3C-Like protease (SARS coronavirus) inhibitor, Papain-like protease (SARS coronavirus) inhibitor.
Pairwise structure-activity
In PASS 2022 the total number of pairwise structure-activity records is 5,174,855 (4,288,195 in PASS 2020), with an average of 512 compounds per activity and 3.2 activities per compound.
Predictable activity types
The number of predictable activity types is 8,565, and 1,957 activity types are in the recommended activity list. The average invariant accuracy of prediction (IAP) exceeded 0.93 for all 8,565 predictable activities, and is over 0.97 for the recommended activities. Depending on the particular purpose, the user may include into the predictable activity list any of the 8,565 activity types using the “Selection” procedure.
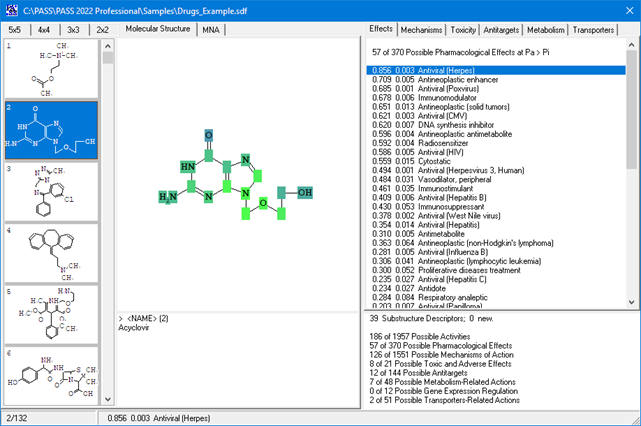
In PASS 2022, the MNA descriptors (for prediction of activity spectra or for adding substances to SAR Base) are generated if structure corresponds to the following criteria:
- each of the atoms in a molecule must be presented by atom symbol from the periodic table. Symbols of unspecified atom A, Q, *, or R group labels are not allowed;
- each of the bonds in a molecule must be covalent bond presented by single, double or triple bond types only.
All other limitations on the structural formulae implemented in the previous PASS versions (only one uncharged component, minimum three carbon atoms in the structure, MW<1,250) are not applied anymore.
If the structure does not correspond to these criteria or the input data contains any other errors, a message about the first critical error will be received.
For a multi-component structure, only the largest component (with the largest number of heavy atoms) is taken into account.
Based on the prediction results, you can evaluate the contribution of each of the atoms of the structure to the estimated biological activity. Select the desired biological activity in the predicted activity spectra by clicking on it; then, each of the atoms of the structure will be colored according to the following scheme:
Light Green Pa = 1, Pi = 0 (atom promotes activity)
Light Red Pa = 0, Pi = 1 (atom promotes inactivity)
Light Blue Pa = 0, Pi = 0 (atom does not generate any signal)
Grey Pa = 0.33, Pi = 0.33 (atom equivocal for weak signal)
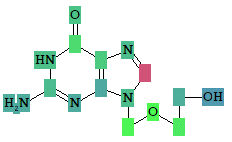
Acyclovir, selected activity – “Antineoplastic enhancer”.
How to cite
How to cite PASS:
Lagunin A, Stepanchikova A, Filimonov D, Poroikov V. PASS: prediction of activity spectra for biologically active substances. Bioinformatics. 2000 Aug;16(8):747-8. Link
How to cite PharmaExpert:
Lagunin AA, Goel RK, Gawande DY, Pahwa P, Gloriozova TA, Dmitriev AV, Ivanov SM, Rudik AV, Konova VI, Pogodin PV, Druzhilovsky DS, Poroikov VV. Chemo- and bioinformatics resources for in silico drug discovery from medicinal plants beyond their traditional use: a critical review. Nat Prod Rep. 2014 Nov;31(11):1585-611. Link
Recent application of PASS and PharmaExpert, an example: In silico analysis of antitumor affects:
Bocharova OA, Ionov NS, Kazeev IV, Shevchenko VE, Bocharov EV, Karpova RV, Sheychenko OP, Aksyonov AA, Chulkova SV, Kucheryanu VG, Revishchin AV, Pavlova GV, Kosorukov VS, Filimonov DA, Lagunin AA, Matveev VB, Pyatigorskaya NV, Stilidi IS, Poroikov VV. Computer-aided Evaluation of Polyvalent Medications’ Pharmacological Potential. Multiphytoadaptogen as a Case Study. Mol Inform. 2023 Jan;42(1):e2200176. Link
GUSAR
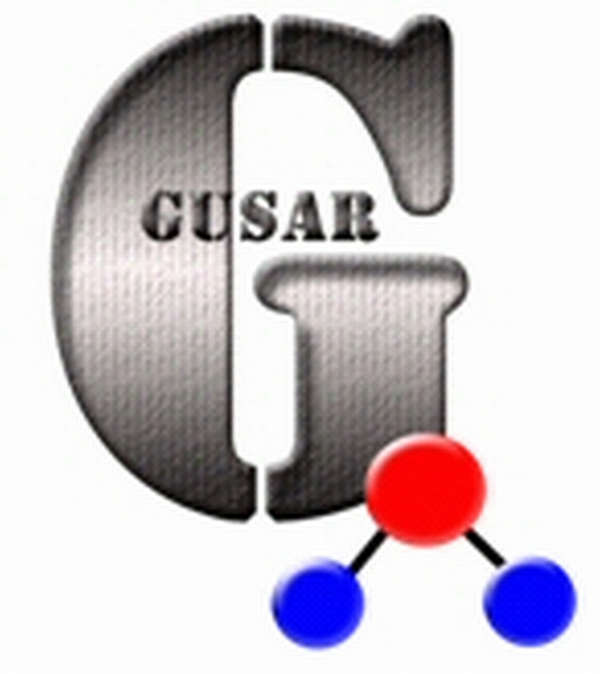
Create quantitative models on structure-activity (structure-property) relationships.
Run your compounds on pre-computed quantitative models
Introduction
GUSAR is a tool to create models on quantitative structure-activity relationships. The acronym stands for “General Unrestricted Structure-Activity Relationships”.The input of the program is your training set of chemical structures and quantitative data on biological activities. The output is a reliable quantitative SAR/SPR (Structure Activity and Property Relationship) model.
GUSAR user interface
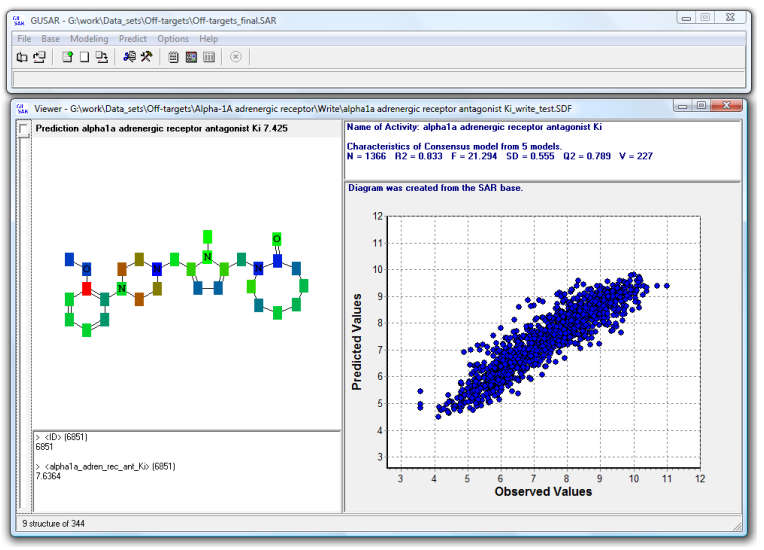
Quantitative prediction of the effect of a chemical compound.
GUSAR features and applications
GUSAR algorithm
The core of GUSAR consists of a unique algorithm of self-consistent regression that allows to select the best set of descriptors for a robust and reliable QSAR model.
Chemical structures are represented by MNA (Multilevel Neighborhood of Atoms) or QNA (Quantitative Neighbourhoods of Atoms) descriptors and biological activity descriptors that are based on the PASS prediction results for more than 8000 biological activities. QNA descriptors easily reflect the nature of intermolecular interactions. Models developed using biological activity descriptors enable to reveal key mechanisms of action of complex biological effects. MNA and QNA descriptors are used to calculate several variables, such as topological length and volume or lipophilicity of a molecule. For further details, see Filimonov et al. (2009), Zakharov et al. (2012), Zakharov et al. (2016).
Precomputed GUSAR models
The GUSAR software optionally includes ready-trained GUSAR models (SAR bases) for predicting certain biological activities. These are SAR bases that can be used with the GUSAR program for predictions on acute rat toxicity, acute mouse toxicity or antitargets (off-targets).
The acute rat or mouse toxicity SAR bases can be used for in silico prediction of LD50 values for rats or mouse with four types of administration.
A quantitative prediction of antitarget interaction for chemical compounds can be done with the other SAR base. The QSAR models for the set of 32 activities (using IC50, Ki or Kact values) includes data on about 4,000 chemical compounds interacting with 18 antitarget proteins (13 receptors, 2 enzymes and 3 transporters).
GUSAR publications
WHAT MAKES PASS and GUSAR DIFFERENT FROM OTHER TOOLS?
How to Cite
How to cite GUSAR:
Filimonov DA, Zakharov AV, Lagunin AA, Poroikov VV. QNA-based ‘Star Track’ QSAR approach. SAR QSAR Environ Res. 2009 Oct;20(7-8):679-709. Link
Recent application of PASS and GUSAR, an example: Evaluation of inhibitors of Mycobacterium tuberculosis:
Pogodin PV, Salina EG, Semenov VV, Raihstat MM, Druzhilovskiy DS, Filimonov DA, Poroikov VV. Ligand-based virtual screening and biological evaluation of inhibitors of Mycobacterium tuberculosis H37Rv. SAR QSAR Environ Res. 2024 Jan;35(1):53-69. Link